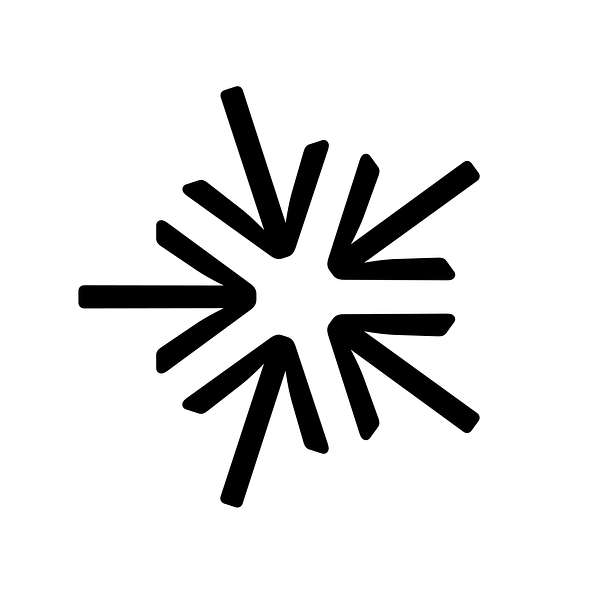
Utopias, Dystopias and Today's Technology
Utopias, Dystopias and Today's Technology
AI Ethics and Democracy: Debating Algorithm-Mediated Direct Democracy and the Democratization of AI
César Hidalgo's "bold idea": https://www.youtube.com/watch?v=CyGWML6cI_k&t=2s
Open AI's plan for AI behavior: https://openai.com/blog/how-should-ai-systems-behave
Arvind Narayanan's talk about AI snake-oil: https://www.cs.princeton.edu/~arvindn/talks/MIT-STS-AI-snakeoil.pdf
Emily Bender on stochastic parrots: https://www.youtube.com/watch?v=N5c2X8vhfBE
Hello and welcome. My name is Johannes and I am the host of this show. I'm here today with Dorothea Baur, who is an independent consultant and a speaker, including TEDx, a writer and a lecturer on ethics responsibly and sustain, um, responsibility and sustainability with a specific focus on tech and finance. She helps companies align value with values. Okay, um, I'm really happy to speak to you today, Dorothea. How are you doing?
Dorothea Baur:Thanks for having me joins. Absolutely, my pleasure. Great,
Johannes Castner:great. Well, let's talk about the, the topic today is, uh, democratization of ai. It's a, it's a ethical discussion, but, um, focused on, uh, around the topic of democratization of ai. And, uh, uh, could you please start by telling me what, what, what do we generally mean when we talk about democratization of AI?
Dorothea Baur:Well, democratization has become a popular term, not just applied to ai, but for basically meaning giving people access to.. And so that means making it democratic or democratize, it means, uh, spreading out AI so that anyone can use it. And that's how democratization of AI is used, and I'm not a big fan of that use of the term as I might. explain later. Yeah. So
Johannes Castner:you've written an article actually that, that, uh, that says, no, we do not want to democratize ai. Could you, could you say, uh, a little bit about that and why, why are you so opposed to this term of democratization, AI
Dorothea Baur:Well, that was, of course, titles are always a bit provocative and saying, no, we don't want to democratize. If you take it in the meaning that I just described, like, no, we don't want to make it possible for everyone to use ai. It might come across as elitist and exclusion exclusionary, and that's totally not what I, we mean, but in that piece or in general. Um, I, I dig into what. Democracy and democratization actually mean, and I am convinced that democracy does not boil down to just, uh, including people or giving people access and that it shouldn't be used. Uh, especially because democratization of AI is often used by commercial actors. So when they say democratization of ai, it just means we want to. So, you know, there is really, there is no moral quality in it. There is no justice component. It's just a misuse of the term, and I think it's strategically used in order to overcome prejudice and skepticism towards AI and in order to legitimize massive rollout of ai. So, . Yes, I want technological progress in general to be accessible for as many people as possible, but a, not under all circumstances. And B, that's got nothing to do with democratization,. Johannes Castner: Okay, well, Why? Why does it not have to do with democratization? You could imagine that, so, so you could imagine, right? So that, that, that power, right? So democracy has something to do with power, right? You could imagine. If, if everyone can use ai, say the way that currently only Google and, uh, you know, a few specialists can, and even, you know, when I learned to, to, um, well use AI to, to apply AI to various problems, uh, when I first learned that I was quite technically involved, right? So I had to learn linear algebra and calculus and a lot of things. So, so you're very focused on these very highly technical. And sometimes you might be missing the, the substantive picture. Right. You know, uh, uh, in, in, in this process because your, your, your focus is entirely on the technicalities. Right. So in that sense, for me, it, it, it has freed me up to think about much more. Um, Much more about the, uh, the applications themselves and, you know, and, and, and the ins and outs of them. You know, for example, uh, you know, the, the, the problem with, um, collaborative filtering where it always recommends things that are already popular, right? So why do I need to have a recommendation about the Beatles, right? I don't need, I don't, nobody needs that, right? Everybody knows the Beatles. So in that sense, you can start thinking about those kinds of things that are much more substantive and quant qualitative rather than, you know, technical. And isn't, that actually isn't that beneficial in itself just because it allows you to think about the benefits and the costs much more. Well, but if I understand you correctly, is what you describe as your approach to learning to understand ai. So you had, you dug into, you know, linear algebra, which I never did after I was released from high school, I, I, I never touched a math book anymore or I did in, at university, but not much longer. So, and, and so this felt empowering to you? It. It empowered you to better understand the tools or that you're confronted with as a user on the internet, and you were probably able to develop some. tools yourself, et cetera. But mm-hmm. again, I mean that's got nothing to do with democratization in, in terms of making it democratic. But, um, not many people will take this approach to AI and not many people will dig into a linear algebra. Right. And it's not what people who say, oh, we are democratizing AI; ChatGPT is democratization of AI because everyone has access to it. Yeah. So it's not. The evangelists usually democratization of AI is used by people who call themselves tech evangelists, which I also find a very weird term that's like a religious component, a political component. You know, that's just take, they just appropriate words from whatever context they deem helpful for, uh, enlarging or increasing. Power. And so that's Oh, but
Johannes Castner:that's a, okay, so, so increasing the power, right? My power as, as someone who knows technical things, right, is decreased and it's given to the people who don't know them. Right. So, and that's what I was trying to allude to. So, so I had to learn linear algebra and go through all of these things. And not everybody can do that. Not everybody has a time. Not everybody has the, some people just will give up, right? They won't do it. Right. It's, it's technically, it's difficult and so on. You have to dig into it. Yes, it empowered. over others, right? Because those who, who then can't understand linear algebra will then sort of be exposed to AI sort of in a, in a much more passive way, right? So now it enables these people who don't want to learn linear algebra to still use AI just the same way as I do, right? So it it, it levels the playing field, if you will, and why not? And in a way you could say, AI is a power, right? So like, so, so let's, let's say, okay. Democratic systems as we know them, you're right. And it probably has not very much to do with that, but you could say that AI is in the, in the, in the hands of those who can. Learn linear algebra, uh, be hired by companies that have large number, a large data sets, right? Because without the data set, even if you learned the linear algebra is useless, right? It's, it's not gonna do anything unless you have the data. So now, now suddenly, like, I think this democratization thing, there's a little bit of a, of a kernel of, of, of. Truth there because you can say that AI, in a sense, is a powerful decision maker, right? Or it it can be. And so now if you are allowing more people to use this powerful decision maker who can go through and zift through all these data and make sense of it, and then make a much more rational decision for you, isn't that in some way kind of in the spirit, even not in the letter of, of the concept of what democracy is?.
Dorothea Baur:I could have interrupted every other sentence of you, but I'm sorry. I'm, I'm a polite person., no. Well, I don't, I need to remember now. It's like, oh no. Oh, no, no. This is not true. No, but you know what is true? What is absolutely true? It is. making it more egalitarian. Mm-hmm.. Mm-hmm., that's what you say levels the plane or the playing field somehow. Yeah. Yeah. The playing field. And, and that's really important and that's what I acknowledge. I mean, whenever I say democratization of ai, I use quotes. Yeah. So even recently I found myself commenting on someone's post on LinkedIn, but listen, um, it was an interview with an expert who said she does not see the value of. Text machine that generates synthetic text about ChatGPT. She's very critical of that. And, and I, uh, and someone posted, like the journalist posted the interview and I said, I agree with all the many statements of that person, but I disagree with the fact that there is no value. It because exactly ChatGPT allows people to write good text or. Sufficiently good texts. No, but imagine dyslexic people, for example. Um, of course now they have, you know, you already have, um, how say, uh, spell checks, et cetera. But in general, it, it empowers people. It gives people a voice, which seems to be their own, but okay, that's where we already enter into premise. But it, no, but I mean, it lowers the barrier. To, to access to the world of written concentrators. And I'm not asking questions about the purpose and meaning, but it is, it has an egalitarian component and especially the fact that it is free. In the case of ChatGPT, we will see where it develops, how it develops and how they will commercialize it. But yes, egalitarian and other thoughts., you said something. Wow. So now people, uh, don't have to go through the same hard, you know, school like I did with linear algebra and, uh, they get empowered by using ai. And then you said, if I remember correctly, that allows 'em to make much more rational decisions. I mean, like a red flag and you, you frame AI as a powerful decision maker. But, but that's exactly where I struggle. I'm Okay. There is a, a distinction from, um, a guy, I think it's Narayanan who writes about AI and snake oil, where he say there are like three types of AI applications. Like one is. Analytics, you know, like parliaments scanning and where we make a lot of pro progress. Then the other one is whatever, something, and then like the, the most complex case is, uh, dec, um, decision making, like predicting. I think the second context is something like, um, judgment, where you use ai, for example, to filter out hate speech online, which is already more complex. So we can be sure that when you apply AI. Necessary for decision making about the future, but about is this parliament? Does it show a healthy body or like, uh, does it show something, uh, to be worried about? That's where AI is getting better and better. I mean, it's still not there yet, but in the second context, it's already more difficult because you trust AI to distinguish hate speech from non hate speech. And we all might have different opinions about what constitutes hate speech. So, there, it already becomes a bit more delicate. And then the third one, you know, prediction where it is like based on big data that says people like Johannes with the same characteristics who used to do this in the past are likely to do this in the future. And that's why we will, you know, give him this in this conviction, uh, for jail or for crime he committed or did not commit. So that's why it becomes much more problematic and. Right? Yeah. The recidivism case, and that's exactly what I mean. That's when you say AI is a powerful decision maker. Mm-hmm., I say powerful decisions is the context where you least should use ai. It's most dangerous context for using ai. Low stake decisions like recommendation algorithms. Whether you get a recommendation for the Beatles or for the stone doesn't ruin your. Okay, but high stake, like powerful decisions about, you know, jail time, about access to social services, financials, et cetera. That's why it becomes really dangerous. So,
Johannes Castner:no, but, but wait, wait, hold on. Why, why do you say that? So, so there, there, I have a, uh, a, a question for you there and, and with re regards to that, , I know it's, it's often, you know, these, these, these high stakes situations is an interesting case to me and nimi. We, we often say, okay, so let's say an ai, uh, an algorithm makes the wrong decision. And we will say, oh, you see, there we go. It's bad, right? But, but the thing is, let's, let's apply this. Let's, let's, let's find a context where this might actually flip. So suppose you are in Alabama and all the judges are demonstrably. The juries that you're gonna find are most likely racist because they're gonna be admitted by the judge. And the judge has a sort of, a gate is a gatekeeper as to who gets in, right into the jury. Jury. They might just pick racist juries, right? So, so now let's, let's look at that case and say, well, let's introduce a slightly biased algorithm into this and say, and then ask. And then ask the person who is up for recidivism. Or for the discussion of whether they should be released from jail, whether they would like to be ju judged by a, an algorithm that was based in, built on the Silicone Valley, that might be a little bit biased against them, or maybe even a, a lot or a racist judge, right? So these are the options, right, because often. The, the options aren't as perfect as you might want them to be. You might rather say, no, I want to have a fair judge, a fair person. And then you compare that against the algorithm. And maybe you're right, under certain circumstances, the fair judge might be a better, better decision maker than the algorithm, but it's not always the option, right? So, so we have to compare the algorithm against the alternatives. and, and when you do that, well, I mean, sometimes I would, I would prefer an algorithm sometimes,
Dorothea Baur:you know, in these cases, no, I, yeah, sometimes. Sometimes. But it could also be the opposite. You know, you are whatever, uh, in front of a jury that, you know, uh, just totally different scenario. And the thing is just, I mean, people are biased. Algorithms are also biased, as you just said. It's just on different scale. People are biased in a, it's not always as easy, like, uh, of course you have racist tourists that will tend to always, uh, uh, you know, uh, give harder sentences to people of color than to, to other people, et cetera. Of course, you have these. Textbook cases of, of straight, uh, forward racism, black or white. But there's also another type of bias in humans, like being moody. Uh, today I'm giving you a hard sentence because I've had a fight with my husband the night before, whatever, or, uh, uh, today I'm really nice to, because you remind me of someone I really appreciate, uh, or, you know, so it's not, it's not, it's not that easy. And then knowing that people are bias, Why does it justify to embed biases into systems that then take these biases as the standard decision making rule? Because whereas it doesn't justify tomorrow,
Johannes Castner:I, I agree that it doesn't justify it, but you could say, When that, that in the case of the algorithm, you can, you can, over time you can say, uh, you, you can discover the biases and you can say you, you can correct for them, and then they will be corrected once and for all. Right? Whereas a person is, by the way, also this, this, this attaches to the, to this idea that, that I read a lot about was explainability, right? Humans are absolutely unexplainable. Let's start. Right, like humans are not explainable, like you said, they could be a mood, mood swing, or this or that. So AI currently is also not explainable. So we got one tomato, another tomato. Um, but, but it is at least in principle, Possible to improve the AI in a, in a systematic manner, where you can say, we found that last year this AI uh, system, this algorithm has been biased in, in that it, uh, and, and that it had a say, uh, a higher, um, a higher uh, rate of. of, uh, rejecting the, the, the, the proposal that say, uh, black people are let out of jail, then, then it is warranted for the, for the black recidivism rate, let's say. Right? So you have to compare it to the actual recidivism rate of, of, of black people, uh, say. And then you can say, well, this algorithm is biased, and that it, you know, on average gave it actually, uh, pretended or acted as if it, if as if this rate was actually higher. Right. So that would be the bias, but then you can say that and you can, you can find it out and then, then you can sue the company or you can then say, well, we have to improve the algorithm, and they improve the algorithm. And then it's improved compared to before, right? Where with humans, there's no such thing, right? There's no way that you can systematically improve human decision
Dorothea Baur:making. You say humans. are not explainable. And, and, and that's true of course, but I will always be able to justify my actions unless, well, even if I did the minute wrong state, I will tell you I was drunk. I can't be held accountable. But so, and that's the thing, humans are accountable for their actions. If a judge systematically violates rules or whatever he needs, or she needs to fear to be, you know, fired or put in jail, an algorithm has no conscience and faces, no consequences. So of course, this is about liability of the software provider or the liability of those who use it. All these legal questions. So first of all, Humans are accountable as opposed to algorithms directly. They're not accountable. And second of all, if you say, oh, next year we will find out that unfortunately we have been using an algorithm that was totally skewed and that was totally unfair. How many lives have been affected? How many, how many people have spent that year in jail? So it's no, it's absolutely not. We can't do away with people in especially what I also struggle with, and that also leads back to the whole democratization. It's a. Binary review of democratization because it's always, um, like algorithms are always about the outcome. So it's either a yes or no, or jail or no jail, et cetera, but when in fact, democracy as much as, uh, a process in court. Is a lot about deliberation. We exchange arguments, we form opinions, and while I'm talking to the person in court, they form their opinion. They listen to me. So it's, it's a very discursive process and. Delegating such processes to algorithms, speed in court, or in democracy or, you know, uh, in democratic context, or labeling it as democratic is just getting it all wrong. Even more so, because algorithms, as you know, or like machine learning is notoriously black-box style. So yeah, that's, it's, it's really, it's, it's, I think it's the. imaginable context for using such
Johannes Castner:tools.. But even if so, so let's say, you know, drive, let's, let's move away from the recidivism for a second and go to driving. Right? So if it, it's true that even in, in, if, if a car, if an say an automated car, self-driving car crashes into someone, they're not accountable. And that's a huge problem. And, and, and I agree with that. That's an accountability issue. Although the accountability, you could also. Well, it is accountable in the sense that we'll improve it now because we now uncovered its mistakes, right? Whereas with the person, if you punish them, you don't know that they're gonna improve because of that, right? So with an algorithm, you can literally say, we're going to improve you now, and that's the consequence you're getting, which is. Unsatisfactory to us because we like to see punishment, right? This is like something psychological. I think actually this is not, not necessarily, uh, irrational or reasonable even. But, but then, um, but then, you know, so in the case of of self-driving cars, we now know that self-driving cars are invariably safer than the best drivers out there. They crash less., right? Yet, every time that they crash, that they do crash because every once in a while there will be some conditions that hasn't seen some kind of flare, something that hasn't been built into the or. The data hasn't been. been there, and then it will crash. And then we will freak out, right? And and much more so that if another drunk driver crashes somewhere, you know, so what? It's just another DRI drunk driver. I find that in some ways irrational. Now, there is a rational component on that, which is this accountability issue, and then you know who you gonna sue if this thing is gonna crash into you in damages your car, right? You have no one to sue. You have no recourse in a sense, unless., you'll find out a way to do that by saying maybe this algorithm was developed by x, y, Z company and they can be liable or something. Somebody has to be liable. I understand this liability issue. Um, but, but I think that the irrationality of the freakout is much worse than, uh, what just this liability issue alone can account for.
Dorothea Baur:Yeah, I know, and it's been a while since I dug into that, you know, self-drive cars debate because it has been overshadowed or overtake by the whole generative AI recently. But there are a lot of arguments on why, you know, even though the accident rate of, um, self-driving cars will be much lower than of humans, uh, , it is still morally justifiable to stick to humans as drivers. And I, I can't really remember all the arguments anymore, but of course it has to do with accountability. And also a lot of it, a lot of the criticism about self-driving car has to do with vastly exaggerated claims about what they are able to do with the fact, you know, they have been promised prematurely to be all over the place. Uh, and you know, so it had, I think, The criticism refers to that and to the fact that, uh, they're actually only. Uh, safe at the moment in a very narrow context, the reason, so something on TV where they use robot taxes or robot caps in, in, in Phoenix and they say that's a good place to use it. Phoenix, Arizona has broad streets and a lot of sunlight so that these are ideal conditions. So I think, um, a lot of criticism also, uh, around self-driving. All also refers to, to, to kind of, uh, untruthful promises or exaggerated like hype, et cetera. But, um, you're right from, uh, utilitarian perspective saying like the greatest happiness of the greatest number, uh, you would have to probably eventually, once they're ready, prefer a self-driving car. humans. Yeah. But I'm, I haven't really formed a final opinion that I always, of course, am a bit critical towards, uh, hardcore, utilitarian, uh, approaches. I'm more of a can if I have any leaning. Johannes Castner: But, but that's interesting actually. The thing, uh, I'm, I'm not sure with respect to can, I'm also not a utilitarian though, um, because, um, well, I, I don't believe that, uh, a Western standard of any sort should be, uh, should be hoisted upon the world's population, which is often what people think. You have a good ethical system, maybe utilitarianism is a good system, but it's not. The only system, and some people might find it vastly unjust because they follow a completely different ethical system that is just as legitimate. So this is my problem with, with these kinds of, uh, schools of thought that, uh, that they shouldn't be applied, uh, you know, widely, they should be applied to people who are. They should be applied to, to other Utilitarians, utilitarians, and that's it. Pretty much. But, um, uh, but, but the site, you know, that's, that's my own view on that. I, I, myself, I'm much more inspired by, um, by, by the, um, uh, by the capability approach that was put forward by SEN and, uh, and, and Mar Martha Nusbaum, um, which is actually all about, you know, involving the. Or it's at least in part, involving the people who, who, you know, who are affected by decisions, uh, whether they are done by AI or by humans. I think actually the distinction there is not that important. Really. It's, it's about decisions really. I think these ethical systems, they're not really about who makes them. That's kind of a new problem that hasn't been addressed by these systems, I think directly. But this, this question of who makes them is an interesting one. Still, nevertheless, and I do still. I mean, I, I feel like you haven't convinced me yet quite that, that, uh, that, that, you know, it isn't even, even though you, you're right about, so, so this, this thing that, that some people's lives might be damaged in the process. It's the same ca same as also true if humans badly make decisions about the recidivism, say for example, for one year, except that you might never know, right? So, so the, the, the, the good thing. algorithms is that you can, you can actually say that they were biased. You can say that they have these in that kind of property and that these properties are, are problematic and that they can be improved. And then, then you have a sort of, you know, that they're in a way self-documenting. You can, you can figure out what, what is going wrong with them, right. With with humans, you know, even though they're not, explainable, they have this lesser, you know, explainability of course would be very nice, but that is lacking across the board. Humans are not explainable, as I said before, and I think this is why, why the standard is so the, this demanding standard of them being, having to be, uh, explainable to me doesn't make that much sense. Because I think that we should compare, always compare it to the next thing. So in the, in the self-driving car, A scenario, we can make a nice little comparison and say, well, how many accidents happen by human, by the average human driver, by the best human drivers we can compare it to, to something. Right? Whereas with the recidivism algorithm, for some reason, we never make that comparison. We say, oh, this algorithm is biased, therefore we should have humans. No, I don't think that follows . So that, that's where I don't, well, I.. One thing is that we are still early days, you know, of the whole discussion also about bias and algorithms and everything that has come to light might only be the tip of the iceberg. So we have now known unknowns, but we still have a lot of unknown unknowns and you know, algorithms or the whole AI debate., um, really has highlighted a lot of social injustices, uh, due to its meticulous statistical pattern recognition that otherwise we would not have dis detected. Yeah, so that's really helpful. It's just. Painful to go through the process of, you know, having it applied to people and making unfair decisions or hurting people, you know, whatever, the whole bias thing. But I still think, um, you know, there are like, there's, there are a lot of unknown unknowns and, and that's, That's what also bothers me. And when you say, so when we develop a self-driving car, we have a benchmark. You know, you know how many accidents do human driver's cars? Mm-hmm., how many accidents do, um, self-driving? Cars. Cars. But we can't really trust self-driving cars to be there because they, they, they have not been released into the same unpredictable, crazy. environment, uh, that human virus have been released into. And, and so all the development of, uh, you know, technology or, or of tools that we use of engines has always been done under the premise. How can we create engines that are, uh, best suited for human use and that, you know, don't exploit human weaknesses, but rather mm-hmm. Difficult to be abused, et cetera. So it was always, uh, development of engines for human users. And now, you know, we are having a, a totally like separate, uh, thing. So we, we, but with this
Johannes Castner:democratization complex. So that's interesting though with this democratization. If, if we call it, uh, under quotation marks, you could say that an algorithm is sort of an extension of a human right. You, you could think, think of it that way. And so then what we are really doing is we're just kind of. giving more, uh, the ability to, to look at a lot more data to a human being. Uh, to a human being in a way. And, and then sort of deduce things from this, um, large amount of data that a human couldn't do. Right. The limitation, because algorithms, as we know them, they literally come from science, right? They, they, so we started growing regressions, and we didn't call it algorithms. We didn't call. We didn't call them ai, we called them statistics , and I think we should still call them that actually, you know, it's a trend. It's a nice word, ai, I guess. But, but really we've been doing that in order to find out things that we ourselves couldn't, right. And in, in, I guess in that way, they., um, algorithms are, are based on a sort of scientific way of thinking or scientific way of, of dealing with the world, you know, using data and, and so on and not crazy hunches or, you know, the belief in ghosts or something like that, that might drive human decision, right? So we are, we are going, we are, we are allowing people to be super powered by, by something that's based on science or a scientific approach,. Dorothea Baur: In the way. I mean, honestly, you say super powered. I'm like, oh no, no., no, because honestly, that's that's so much., you know what we've learned from the enlightenment? Mm-hmm. like 250 years ago, Manuel can saying have the courage to use your own understanding where he told people to liberate themselves from the shackles of gods and mm-hmm., monarchs, et cetera. And so before we used to be, you know, dependent on Gods will or on the kings will. And yeah, we didn't make an effort to understand our surroundings. and you know, we didn't have the courage to use our own understanding. And then we had that breakthrough, the enlightenment, which set in motion, the whole, you know, everything, the whole progress, including democracy, democracy defined as self legislation. Give yourself your own laws. Well, that's people, that's all you create. That's a, that's a, that's a, a Greek concept.. Dorothea Baur: Yeah, sure. But I mean, it had been dormant for a long time. And then after, you know, after the enlightenment and so, so, uh, by. You know, the, the courage to use our own understanding was a pre-condition, uh, for establishing democratic societies where people were able to contribute and participate and, you know, where the com community of moral agents and patients was subsequently, right, extended, et cetera. So all of that. So now if we delegate, if we let ourselves. Superpowered, I even, I can't even pronounce that word.. Let ourselves be superpowered and understand this as an improvement. We delegate our whole responsibility. No, no, no. That's our freedom to, to machines. So well that that's where the day, that's where I, where I agree with you. We should not do that, but, but we could. so I think there's a difference between doing that, sort of automating our thinking, uh, and extending our thinking, right? So, so we could say, we could use ai, this is a possibility. We, we not, not, we often don't do that. Maybe we're lazy and therefore we wanna delegate the whole thing on it, right? So people sit down with chat, G p t and let them let Chad g p t write the book. Uh, what, what's the point of that? I don't know actually, honestly, there's no point to me in this, but, but instead, if you could, . I, for example, my, my, my wife went to a French school and so she, she learned how to structure an essay really well, right? So let's say I, I didn't go to a French school and I didn't learn that, and in fact, I didn't go to school at all as a child. And so I, I started much later with my educational approach. So I have a little bit of a tendency of having an issue with structuring an essay, for example, right? So I could use, I've not done it because I could use in. use chat g b t to just give me some structure, give me an outline, a structure, a beginning and end in a middle, and so on, right? And then, but, but the thinking really comes from me, right? So, so it's not to say that I'm going to delegate the whole ench to, to, to the ai, but I let the AI help me with my weaknesses so that I can actually think better. And, and so, so a regression, right? Why did we invent during the enlightenment, we invented actually everything that we. That, that went into ultimately building ai. Right. AI is basically a fancy regression. I mean, not always, but it's based on similar tools, right? It's based based on linear algebra, and it's based on, uh, uh, you know, a gradient descent often, which is, you know, uh, which is, um, uh, calculus. Uh, and it's, it's based on, on the same basic principles that, that, that we base science. To help us overcome our human weaknesses because we actually, our perceptive organism is actually not that great. We can't proceed all that. No, but.
Dorothea Baur:Yeah. No, but I mean, so there's, there's an irony of course, that the Enlightenment encouraged us to use our own understanding, and we used it so much that we found ways to, to, to create things that have a stronger understanding than that are, that are smarter. than we are in some, some narratives. Yeah, in some, yeah. So it's like, uh, in term we say the revolution eats its children, , you know, it's like, it, the, the enlightened Is it coming to an end as a consequence of its very own strengths? Um. Are we reverting to, to two times of darkness, black boxes instead of the black box of God dictating your fate. Uh, you know, the black box being an algorithm dictating your fate. Well, that just Wow. Uh, uh, a sad remark. Yeah. Well, that, yeah, but that's it. Because we, we were, we, we thought we were guided by God. Oh, why do I get this disease? It's God's will. So, I mean, why am I sent to jail? Oh, it's the algorithm's. Whatever. So that, that's just a sad remark. So I find that very, very fascinating to think about. Then the other thing is, yes, as an extension of ourselves, of course, not in a transhumanist sense, hopefully , it's not what I wanna say, but as an extension. Yes, sure., I can say I wrote this essay with the help of, uh, Ted G p t. I can say I wrote this essay in, uh, in isolation just with a sheet of paper and handwriting. And you, you, you know, are transparent about the process, how you created it, and then people will come to different judgment. Mm-hmm., they might be more impressed if they see the same with, you know, generative AI creating pictures like tele or mid journey. You know, if I say, well, I have painted. Painting by hand, or this is my creative idea, it has a different value. Not a, not a higher or lower, but just it appeals to different judgment.
Johannes Castner:Well, I think art and Yeah. You know, art is different, uh, from. It's different there because, well, no, no text. Also text, also text and art and music and, and writings. Creative. Yeah. I think creative endeavors have bit different than, you know, driving a car, you know, or, or than, you know, making sure that, that, that the person with a, a likelihood of actually. Committing another crime actually stays locked up and the person who doesn't have a high likelihood of committing another crime gets out. Right. That's just, you know, those things seem to be more in the domain of science. I guess you could separate between these two domains, you know, a creative one and a and a, and a science oriented one. Okay. And then you could also say, Yes, we should remain critical of the AI and, and, and, and keep increasing it and actually don't turn off our brains, but to let it help us with our brains. If we do that. Is it not democratizing? It in a sense, but then,
Dorothea Baur:but yeah. But then please treat AI applications like a, a, a, a car that's run by algorithms instead of your hands on the steering wheel. Mm-hmm. treat them the same way you treat a calculator. Yeah. If I do a math, uh, thing using calculator, I'm responsible for the results. Yes. You know, I can't blame the calculator. So, but then really make the algorithm a personal property of the user. where he or she has full responsibility. Yes. Uh, and that's something totally different. So if I then publish a text, back to text, but based on chat g p t, because there are also, you know, tendencies now to, to, to allow people to shape their text, uh, synthetic text generation programs and to add their own world use and their own language or specifics of their own language. Right? Right. So you can customize AI applications so that they really represen. you and what you would want them Yeah. To be, or how you would want them to decide. And if we go down that road, then it's totally my responsibility for every single word that is there. Same with a car, if you know mm-hmm., if I decide to use a self-driving car, whatever. And then it's really, it's not empowering, not just. It's, it's really like putting full responsibility on the human. Yeah.
Johannes Castner:Yeah. So, so, so you extend the human, you augment. So I like this, this, I like to call ai. I, I like to use this, this abbreviation to, to mean augmented intelligence rather than artificial intelligence. Because I don't think these are intelligences of their own at all. They're just mathematical models., but they can augment our intelligence similar to a calculator. I like this analogy to a calculator. Very much in the beginning, what we did in math courses, we said, oh, you can't use a calculator for the test. I think that's silly because. You know, what is the test for? It's supposed to test you how you operate in real conditions and in real conditions. You'll have a calculator, obviously, and in fact, we shouldn't learn how to calculate 365 plus 782 anymore because a calculator can do that faster than we can. What we should instead learn is how to, how to actually, uh, think algorithmically and how to think as a programmers or, or something like that, or programmers of algorithms, right? and similarly maybe for writing courses, writing courses now, in my opinion, should incorporate, um, ChatGPT instead of uh, saying that, you know, you have to write it without it or something like this, or, you know, we test you whether you used it or not, and we're gonna try to back out whether you used this thing or you didn't use it. But instead, I think we should assume that people use it and now we should teach them best practices on how to use it and how. You know, plagiarism and so on, right? How to avoid plagiarism, all of those things. I mean, that's, I think, you know, as we go forward, we should incorporate the things that people are going to use and assume that they use them and help them use them better, instead of just pretending that we are still in the world without them.
Dorothea Baur:Yeah. No, I mean, I'm absolutely, uh, I, I agree with you in that regard. It's just that, um, I, I'm not a cognitive scientist. I'm not a linguistic expert, so I, I'm not sure about, uh, whether there is any danger or risk, if you want, of people losing really essential skills when we, uh, kind of, um, deprive ourselves of the capability to write our own text, et cetera. I'm, I'm not sure you know, how the brain works and, you know, they're saying that every augmentation is an am. I mean, how many people are still reading maps? So if your gps don't not work, you're just crash into a wall or you're drive into the woods because you're just, you know, you can't navigate anymore without it. So, um, you know, always need to decide there. But of course, I mean, why. Ignore it. I think there need to be different contexts with different uses of ai and maybe there should also still be some context where we say, let's train to work without ai. Let's, you know, compare what we create without AI to what we create with ai. So, you know, we need to. Complement different users and is it augmented intelligence or is it complimentary intelligence? It's different skills. It's augmentation, but it's also complementing, but it's certainly not replacing. Yeah. So if,
Johannes Castner:if we augment, so if, if we do use, if, if we do have these machines to augment our intelligence, if we have access somehow to the trained models that are trained on the data that we don't have access to, so, so, Um, you know, basically if we're moving from a world where Google and Amazon have all the data and all the compute power to one where we suddenly have compute power and indirect usage of the data, isn't that a form of democratization to come back to that and, and isn't that, you know, this augmentation makes us all more powerful, right? Isn't Democrat, isn't this concept of democratization in some ways? meant to make the average guy more powerful relative to this one desk, desk pot that sits there and is the most
Dorothea Baur:powerful. I think it has potential to make people more powerful, but it, uh, but that's not how the tech evangelists use it. That's what they mean by it. It, uh, and, but it, in theory, in an ideal world, it has for. In a hypothetical world, it has potential to make people more powerful, but it uh, To, you know, uh, power, uh, implies responsibility. So for me, it involves you to, to become, become more responsible. You cannot become more powerful without becoming more responsible at the same time. So, and then I also think we must not overestimate, uh, People, you know, if, if, if even now, experts still, I mean, you know, many things in, in machine learning cannot be deciphered and are, are not explainable. You know, what can leverage user do with, uh, their own dataset that's maybe, you know, attractive for? No offense, but nerds like you, . Sorry. No, no, that's fine. But no, no, I think you're a self-proclaimed nerd.. Yeah. Uh, so, um, it's a compliment. Um, yeah. So, but, but, but, but for like 99% of the users, you know, they just. Blindly trusted. It's the same with the whole privacy discussion. You know, we're saying we're empowering people, we want informed content, informed content on, on, uh, terms of service, et cetera. Yeah, of course you could inform yourself by reading that, uh, stuff, but no one does. And, and so I think it's not, it just doesn't, uh, correspond to the reality of how humans, uh, are.
Johannes Castner:So we, we've talked a bit about the so-called democratization of algorithms. Maybe we can now, uh, talk a little bit about algorithms four and maybe against democracy. Again, how can we use algorithms to enhance or improve democracies. Can we do that? And we know for sure, I mean, maybe we don't have to talk about this because we all know that algorithms can be used to destroy democracies. It's a, it's a famous case. So I had a conversation before I talked, uh, with you, uh, with chat g p t about this. I ask it, you know, what, what do you think about democratization of ai? So I I, I, I wanted to know , and it, it, it has obviously like to no surprise, a. For algorithms, um, helping democracies. And, uh, it, it really has, it's, it has a hard time with, um, you know, with all of these, uh, you know, criticisms of, of algorithms. It turns out I, I guess I'm, I shouldn't be too surprised , but, uh, but, um, yeah, let, let's, uh, I guess the most spectacular case that I know of, , um, uh, democracy of, uh, four, four democracies or, or is, is an idea that hasn't yet been implemented, but that was, um, I guess spurt by a lot of the issues that we see with democracies.
César Hidalgo:Is it just me or are there other people here that are a little bit disappointed with democracy?
Johannes Castner:A lot of people are actually losing faith in the institutions of democracy and because of that, um, and a professor I once had at MIT, I took a course with him. Uh, name is, uh, César Hidalgo. He came up with. With a, a, what he calls a bold idea. And that is, and so I'm gonna briefly, you know, just, uh, briefly, uh, summarize it so that we can then discuss it. So the idea is basically, Um, to move to a direct democracy, but that is algorithmically mitigated or helped say. So the, the, so really briefly, the idea is that everyone can vote on every single bill. So the bills are still proposed and argued by the senators or congresspeople just as they are today, at least in the version zero. He later then also elaborates that there's a possibility., even the writing of the bills themselves could potentially be done collaboratively between 300 million Americans. But, uh, for example, in America, uh, or in, in another country where however many millions of people there are, but the idea would be that everyone can vote on every single bill. I guess that's the case in Switzerland already. Is that, is that right?
Dorothea Baur:Well, not on, well, in theory we could vote on every single bill, but the usual process is that, uh, the. Drafts bills, mm-hmm.. And only when, uh, there is a referendum, when a certain amount of, uh, signatures are collected, we are asked to, uh, uh, for example, because Parliament says we want to have this and this bill, and then someone, uh, launches, referendum and collects votes or like signatures, then the population is being. or like direct initiative. If people say we are not happy with an issue and the Parliament does not address it, you can also collect votes. You need more right than for the referendum for direct initiative, and then you can launch an initiative. But that's always aimed at changing the Constitution. That's not just a a, a law. It's. Constitution, like the highest level.
Johannes Castner:Oh, incredible. So that's, this is different from the proposition system that we have in the us. In the US we have this kind of proposition thing. For example, legalization of marijuana, we can bring that up and have a proposition and then vote on these kinds of things. Mm-hmm.. But, um, but, uh, and this is only for each state actually in the US it's, it's state by state. But, but, so this idea here is that builds are brought up as tradit. Um, by, by, uh, by Congress people or senators. Um, at least in the first version later on, the idea would be completely to go to a direct democracy where even the bills themselves are deliberated and brought forth by citizens. But that's, uh, let's put that aside for a second. Let's say there are these bills and magically come out of parliament and then we've all sing vote on every single point. And so, so not only the build itself, but even the points that it makes within it, right? So we can vote on little amendments and changes of the bill and so on, right? So this is the idea, the, the problem, the obvious problem with that was pointed out by, I think it was Democratis in, in Greece, that, that this would be, uh, impossible to scale. There is a huge cognitive overload with humans. We cannot read, you know, bills are very, very long texts, um, that we would have to all digest, each of us, and that this is way too much to, to demand from citizens. Um, so then the solution to this then is that we, we do some of that to train a digital twin or a version of ourselves that is, that represents us. Uh, he calls it a, a little jimy cricket, um, that, that, that can then vote on your behalf after it knows how you would vote. And you can, you can disable it and vote on every single thing yourself, or you can allocate things to the, to the algorithm. So this is the idea to essentially fix. And, and so, so to explain why, why this idea, we have to first explain of course really quickly. Um, what problem does this, does this solve? As with everything else, I think algorithms should always solve a problem that exists and, and not just, you know, be cool or. And so the problem here is the vulnerability that is inherent in, uh, in representative democracies. That is that if we have 200 people representing 300 million Americans, uh, for example, Well, I don't know some x amount of number of people representing some Y amount of citizens, right? Where y is much, much larger than X. When we have, whenever we have this problem, these, these smaller numbers of representatives are extremely vo vulnerable to being captured either by industries or by foreign governments, enemy government. Hostile forces and so on, right? So, so there's, you know, in particular you could think of maybe the Russians helped Donald Trump to be elected, for example, right? Or, or maybe they were involved in Brexit or something like that, right? So then, you know, we have this problem that we know that we are vulnerable, and one of the proposed solutions is to do this direct democracy that is mediated or helped out, helped along by some. Avatar, perhaps that represents you or, I don't know. I think that's a little bit gimmicky, but the, but the point being that a, that an algorithm basically takes over from you as it learns, and if you change your opinion, it will, you can retrain it and you can teach it that you've now changed your opinion and that it should take over from your new opinion and so on. So what is the problem with that?
Dorothea Baur:Well, it's totally, again, it's against the idea of self legislation that we are, you know, giving our. Selves we are, do you know, we, we are engaging in deliberation, in the discourse, and we are crafting our laws. And it's, it's a misunderstanding of democracy as just a mouse clicked exercise or like a outcome oriented system where at the end, you know, there is a, a, a bill or there's no bill, and it totally ignores all the other elements, uh, and the whole system of tax and balances that, that make a democracy wholesome. not wholesome as, you know, whole parliamentary processes. The role of, uh, the, the, the courts, the role of the electoral systems, et cetera. And part of what you describe about, you know, the US and the, the representatives being vulnerable. Um, you know, there are a lot of, uh, inherent problems with political system in the us. I mean, . Every country has its political system with its own problems, but I don't think algorithms are going, uh, are, are good fix for that. And also, especially in terms of vulnerability. Why should the 300 million voters not be, um, uh, vulnerable? Why can you be sure that I'm not being paid by someone, you know, I can earn money by clicking this or clicking that. I mean, it's, you know, you have incentives. It's, isn't there something. I don't know if it's, it's in the, in the crypto, uh, sphere where you can, uh, uh, play to, to how you call it, you, you earn money by gaming or something , you know? Yeah, sure. Uh, people do all kinds of things and, and, and it also totally ignores the role of. Experts in democracies. Not everyone is an expert. And of course, the way you frame it or, uh, they, these people frame it is like the algorithm will be an expert and understand everything, but no, it's not. We know that.
Johannes Castner:I'm not sure about that, but, but okay. It's, it's more, I think, So, so if you think of crypto, actually this is a good, this is a good analogy actually, because I guess crypto is borrowing some democratic ideas or something like that. But, but the, or, or ideas of, you know, this decentralization, I guess the concept is de decentralization, right? So if, if you have five nodes that are vulnerable, you, you are going to have inherently a much more vulnerable system than if you have 500 vulner. Because you'd have to attack 500. So, so you'd basically have to have involved, you'd have to sell this gamification thing or this, you have to pay 300 million, or maybe not 300 million, but let's say in the US if you wanna win some bill, you'd have to pay half of the people who might be voting maybe only. You know, 60 million people vote or something like this, and then you have to literally pay 30 million people instead of paying 200 people. Right. So the, the idea is just simply to make it less vulnerable by spreading it out further.
Dorothea Baur:Well, I, I, I just have a huge issue with that. Worldview of the view of humans as inherently and inevitably corrupt. And every representative, uh, in the parliament is basically, uh, co-opted. So, you know, everyone has their own experience. And of course, I am biased because I come from Switzerland with direct democracy and have different political experience than you who have. Spent several decades in the US with all the tragedies of the recent years. But I just like the, the starting point. There's, it's, there's so much rotten in the state of No. So the assumption is that everyone, so, but, but I wanna just correct this because, but that's a worldview that we are, that, that that's the extremely negative worldview. That's also in the decentralization, um, uh, ideology behind cryptocurrencies is like the powerful actors. All bad and, and, no, no, that's fine. Centralization is evil. But, but, but then we have libertarian anarchy and No,
Johannes Castner:no, no. The point is not that I don't wanna go there. Right. So, so actually the, the whole point is that it doesn't have to be everyone. So, so the whole point is that if you have 200 representatives in the US say, or 400, I don't know the exact number. I think it's, yeah. Doesn't really matter how many, like you have, like some number of them. The, the point is that, that the question is always, how many of them do you have to capture to win the game, right? So it's, the assumption is not that, that, that everyone is corrupt, actually. You don't need that. You don't need everyone to be corrupt. You only need to be to, to have x number of them to be corrupt and the other two, uh, uh, and, and the other, and, and some other proportions being at odds with each other, right? So you need to just capture enough. So that you get a majority, which, which, um, which might not actually be, uh, that many, it could be as low as 30%. So, so even Hitler didn't get elected. Now that's, that's, you know, I don't wanna go that deep into that, but, but even he didn't get elected by a majority of Germans, was that the left, the, the, the, the, the more democratic minded people were split between three or four parties and then just enough of a proportion. Captured by Hitler. Right. Or that's why. I mean,
Dorothea Baur:but that's, that's coalition building, that's part of the political process. And as a, as a citizen, when I vote, you know, every country probably has that. For example, I inform myself on a website where I can, you know, um, do a quiz on my political preferences mm-hmm. and where all the candidates for parties have all the filled in their, you know, political preferences and then say, according to your preferences, you have the highest match with this Can. I'll vote for her, you know? Yeah. So, yeah, of course. And then, or I, and this party suits best to your political profile. Yeah. And then, you know, I vote, you know, there are, but it's coing ways to inform, to
Johannes Castner:anticipate. But it's co screening. Excuse you. Right. It's co screening you. It's saying that, You know, they, they kind of agree with you on 60% of the things rather than 40% or 30% of the things.
Dorothea Baur:But I need to be able to live with, uh, uh, with compromise and, and dissen.
Johannes Castner:But your votes, but your vote could be completely unpromised. Not to say that the system as a whole, so if 300 million people worth to vote, say, or 60 million, or, I dunno, how many people live in Switzerland, right? If they are., and you'll still end up with a compromise because each one votes differently and they represent themselves and not, but, but the difference here is that if you have, uh, candidates, none of which really represent you, your compromise starts already with the representative. Which doesn't represent you
Dorothea Baur:well, honestly, then it's my duty to engage in politics. It's not, you know, and, and as I mentioned before, this like radical, decentralized, participatory slash libertarian approach to democracy, uh, totally ignores important of. expertise. And even though you can say experts are like, uh, one of the biggest, uh, you know, promoters of corruption and they earn too much money, et cetera, you know, this whole anti-establishment talk, just not infr it. Yeah. I'm, uh, you know, they play a role. They play a role. I'm glad that the parliament invites a climate scientist. Absolutely. To tell them about global warming and they listen to it and then they form their opinion, and of course they're biased because some of them will be at the same time on the board of a, of a fossil fuel company, et cetera. But such is life and life. Is what happens mo uh, partially outside of algorithms,. Johannes Castner: True. True. But I do think that we have a little bit of say of what life could possibly also be. Right. It could be different. So, so, so my point is, you know, I do agree with this expertise aspect. of it as well. It's a tricky one because again, you know, like you said, you know, the, the climate change expert can be a complete fake, right? It can say, you know, climate change is way overblown and that's why we see a lot in the US and the expert comes in with a snowball from the street and shows, oh, look at there. If, if there's a snowball here, it can't possibly be global warming. Right. You, you can, You can have a complete clown show and they consider themselves experts, whatever. Some people consider them experts. It can be also co-opted, but that's not really my point. You know, even, you know, it's true that, for example, the EPA and these kinds of systems, they do have serious experts until they get fired by Donald Trump. Exactly until they get fired by the guy that everybody that, that the majority voted in. Right.. That's the problem of the system. I, I don't think I, I don't think you can put algorithms in charge, uh, to, to fix all the problems, uh, of, of the system that has, that has, uh, some qualities and also, as I said, checks and balances between legislation and, and, uh, jurisdiction, et cetera. You know, the three executive functions, et cetera. Mm-hmm., and, and if you, if you. in a context where people do not have any. Habit of direct democracy where you suddenly, you know, put all the responsibility on people to, to vote on every single bill. Uh, you, you might be surprised, and I'm just mentioning Brexit example, what happens. Sure. When you ask, uh, decisions of a country once in their lifetime, suddenly, ah, you're allowed to vote on, on, on, on a, on a referendum or, or whatever it is on law. The strategically
Johannes Castner:place one and
Dorothea Baur:then years later, Suffer. They suffer from their decision because they were not ready for it. And True. You know? True. So I, I also, the expert thing that I mean, is not necessarily, you know, experts being caught, but just like we need experts or like people that dig deeper into issues and also parliamentarians who have certain profiles. Like he is an expert on social policy, she's an expert on tax law, whatever. Absolutely. Um, But this kid still exists, and you can't, you can't democratize that, that, or
Johannes Castner:decentralize that. You, you could, you could think of it as a modular, you could, you know, think of it as a modular system where the court still exists as before. You don't have to change everything at once. Right. You could change a little piece. And so the idea would be, and, and so not necessarily. Um, the, the export part is a good, that's a good point. And, and you, you could think of how to accommodate that, right? Within a new system or, or what, what, I guess what César Hidalgo is suggesting is to simply change this one piece, right? Which is this parliament or house of representative. Where, where, where the only thing we change is how, uh, how., um, bills are voted on, right? Whether they're voted on by 400 representatives or whether they voted on by 300 million Americans, right? So nothing else changes. Everything else stays the same except for this aspect. And then these 300 million can then opt to sometimes automate their vote after they know that it does what they think it does, and it does. They can observe the algorithm for a while without actually letting it vote and make their. Votes and then after some time to sort of go on, automate until they change their mind and then retrain it or something.
Dorothea Baur:Nightmare. Nightmare. It just reminds me so much of a portfolio simulation. I'm, I'm using Robot Advisor to invest my money. Ah, and where you could also do a virtual, uh, a virtual portfolio without really investing and see how, you know, the funds that you chose, uh, develop and, okay. I simulate. Okay. Seems to be a good thing. Uh, now I go live. Yeah, but I mean, it's, it's, so, it ignores the. Cursive and deliberative element of, uh, on, on the whole virtue element of, uh, democracy, of exchanging ideas, et cetera. It's such a black box, isolated approach. Everyone in front of their computer interacting with an algorithm that has necessarily inevitably being trained on bias data that never understands context, that, that, you know, that is, you know, limited the way it is this algorithm. Um, imposed or like served to a population that is very susceptible to misinformation and manipulation and that in, in a, in a highly polarized political climate. Uh, I mean, honestly, I'm not
Johannes Castner:sure this, that's interesting, right? Because to, to add fuel to fire. No, I think what we might want to do there then, you know, you're right, this, right now we have a lot of misinformation in particular, But one could argue that it is actually much more difficult to misinformed people on every single bill than it would be to misinform them about a handful of politicians. And so, so basically what I'm saying here is that that misinformation is made easier to, to, to mislead people. To vote for one guy, like a guy who says, make America great again, or something like this is super easy. Yeah. Because you have this one target. It's simple. Right. It's, it's, To direct your funds toward misinforming people, about two or three politicians of five politicians, than it is to constantly misinformation them on every issue that comes up. So, so maybe the incentive structure now is, is, would, would change with it.
Dorothea Baur:So yeah, you're saying Yeah, the, the, the, the, the, the, the point of attack are like more decentralized and, but, but honestly it'll be just be a fight for the masses, manipulate the masses, and there will be, uh, a war of information, et cetera. I, as it is, as it is already, I don't, but, but as I, yeah, it is, but I mean, as I said, I.
Johannes Castner:the incentive. S you know, coming is part of the problem of democracy, right? If you look at the incentives, like right now, if, um, you know, the incentives of, uh, for people who are not very well, let's say who are not, who, who have, I mean, there's some comedians who actually make fun of it. You know, who, where could this guy, and then they show the politician, uh, get a job, right? Oh, the only place it's in Parliament, right? And, and, and this is, you know, this is where we are currently, right? The, it's just a, yeah., Dorothea Baur: but I mean, it's a and citizens to, to change the system. And, and, and I don't think, I mean, yeah. Uh, the, the algorithm solution is, is uh, what we, uh, is like, uh, what we should aim for. So you're against algorithms entirely in, in government.
Dorothea Baur:Well, no, I mean, if you say algorithms to discover irregularities in, in, in, in, in, um, counting votes or whatever for fraud detection, that's okay. But certainly not, never, ever algorithms for political decision making because it's, it's our, it's honestly, it's, it's our duty and it's our. Uh, skill that we
Johannes Castner:have. Yeah, we, well, we're talking about, but then, you know, yeah. The representatives is, it's like we're still stuck with these representatives who are, you know, that that's the, that's the part I think that a lot of people, Are disillusioned by the current institutions precisely because they can be so easily captured by a few people. Yeah. That I think is, you know, the, the, yeah. Okay. Okay. Well let's, okay, let's, uh, let's cap it there. Um, maybe, yeah. Okay. So, so , so, so I see you're saying that it's good for maybe disin disinformation, keeping disinformation in check, helping with, with fake news detection. Um, yeah, those sorts of things. You are, you are okay with
Dorothea Baur:analytics, but not decision making. Mm, not delegating decisions.
Johannes Castner:Interesting. Yeah. Okay. That makes sense. Yeah. Well, thank you very much. Yes, , that was great speaking with you. Well, thanks for me. Yeah, absolutely. Thank you. This show is published every Wednesday at 5:00 AM on the East Coast, 2:00 AM on
the West Coast, and 10:00 AM in London. If you haven't done so, please subscribe to this channel and tell us what you like and what you don't like by putting a, a thumbs up on videos you enjoy and thumbs down on videos that you don't enjoy so much. And please let us know why you enjoy something and what you enjoy and what you don't enjoy. Next week I will be meeting with Alan Tominey, and we will be talking about large language models in particular ChatGPT is one of those.
Alan Tominey:A large language model is effectively, uh, it, it, it's the where AI is at the moment really when it comes to sort of interacting with, um, with the real world.