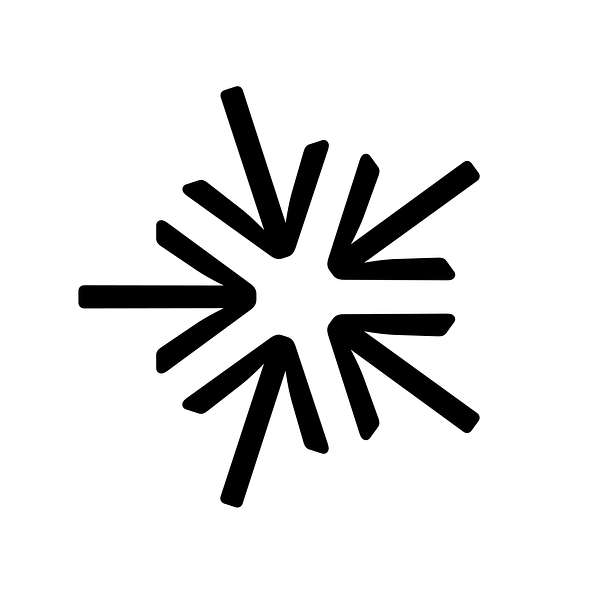
Utopias, Dystopias and Today's Technology
Utopias, Dystopias and Today's Technology
Exploring the Relationship Between Consciousness and Artificial Intelligence
In this podcast, guest Srijit Panja and host Johannes discuss and debate the complex and fascinating relationship between artificial intelligence (AI) and consciousness. They explore the differences between brain-like and brain-inspired AI systems, the unique capabilities and limitations of both human and AI learning, and the potential ethical implications of developing conscious machines. The conversation is thoughtfully guided by Srijit and Johannes's deep knowledge of the topic, and offers valuable insights into the challenges and opportunities of AI research in the 21st century.
Hello, my name is Johanne and I am the host of this show. Today I'm here with Srijit Panja, and, uh, we will be talking about the human brain or the, the animal brain and, uh, consciousness and intelligence, um, as they occur in, uh, AI systems. And, uh, the difference between them and the various designs that might lead to a more brain-like, um, AI system. Srijit is currently a data scientist at Cognida.ai. Prior to this, he was associated with software engineering in data science, wings of Nggawe Nirman Technologies, Infogain India. He has been an Indian representative to the United Nations Development Program, SDG AI Lab., IICP SD Turkey as an, uh, U United Nations, uh, volunteer data scientist, and has been credited for his work on Vilokana, the Indian Covid search engine, featured in news by times of India, new Indian Express Economic Times, the Hindu and Hindustan Times among others, and mentioned internationally in the Nature Journal, which was launched and had been, uh, operational during the first covid wave in India. Srijit's early research interests included computational linguistics and natural language processing. His early work, uh, works as an intern with NeuroAGI Lab IIITM-K Nazarbayev University and startups like Clootrack, B-Aegis were in the area of N L P. He is now inquisitive of brain accurate, not brain inspired neural networks, and is immersed in reading works of Jeff Hawkins at Numenta book, uh, book a Thousand Brains and Neuroscience Resources, mostly hosted by World Science Festival and TEDx among other sources. Okay, so this, it leads perfectly to the first question, which is how do you see the difference between brain-like and brain inspired, um, artificial intelligence systems and do you see. Um, this important difference that I've also discover, uh, dis discussed with, Utpal Chakraborty recently on the show also, um, with, uh, Christian Wilk, um, and, and other, other guests recently. This, this has been, this, you can see it as a sort of series of, of conversations that I've been having around these topics. But I wanna ask you about your, your, uh, research and what you're working on there, and also, um, how you see this important difference and, you know, also, of course, uh, consciousness fitting into this equation.
Srijit Panja:Okay. Sure. Uh, Thanks, thanks for having me here and giving me this platform to like, uh, speak my head out. So like, uh, it's, it's actually a very, I mean, untapped kind of, uh, discussion. Um, so, uh, I, we, we, we see the, we see, uh, AI progressing mostly in, in the, in the, uh, in the direction of brain inspired, uh, internet brain inspired, um, uh, mechanisms and everything. Whereas currently, maybe, uh, uh, some from some time there, we, we are seeing some more improvements in the AGI space and everything. And, uh, we, we are actually getting results in, in that space. So there is actually fundamental, uh, I mean, uh, very, very dis uh, distinctive difference between, between these two rules. That is, uh, when, when this thing started, basically right from, uh, from the start of. When the paper was drafted by basically, uh, by, by Dr. Hinton about, um, back propagation and from there, neural networks and everything started to crop up. So, uh, the essence of that was to captivate how neurons work in the brain and to use that in, in, in a computation model, right? But, uh, so how it is done is basically, uh, uh, in, in the brains as well, in in the brains. The neurons get trained, right? Uh, the, the neural pathways that gets created. When I see something, when I keep on seeing something or keep on getting the same feeling, people, so it, it gets, it, it keeps on getting trained on that. So, suppose I have seen something, or I have touched something for a, for a long amount of time, long enough amount of time. When I touch something from the next, for the next time, I can instantly recognize that this there is this, right? So this kind of a training keeps on happening. Uh, and, and that is how the brains work on a, on a very surface level, we might, uh, get into more, uh, depth and as we speak in the discussion. Uh, so on a surface level, this is the thing that happens. So taking from that, I think, uh, what has been done in the current dual net is to structure it in a way that we have neurons there, but that incremental process, that evolutionary process, process that I should, uh, I mean the, the sense organs or, or the first input layer should ha keep on having that layer to get trained, trained, trained that is not being followed. Instead, we have some calculative methods, right? So in the D replication itself, we have gradient dissent these functions. So we take help of calculus. Mm-hmm. And we basically optimize, right? We, we take a loss function, uh, and we try to minimize that, pull that down, which is, which is a lit, which is a little, I mean, uh, more, more manipulative, not, not exactly natural to be, to be very precise. So that is, that is what I think is, is the main difference, fundamental difference between how, um, the root of AGI is, uh, computational building is and, and how the, the current scenario is in the neural net.
Johannes Castner:So I have to ask you there follow up question because the, the difference that, um, norm Chomsky locates is one where, Where, what he calls it. And I wanna, I wanna quote this, uh, directly because it's, uh, it's so beautifully, um, beautifully formulated by him in the, in the New York Times. I think it was. He says, the human mind is not like ChatGPT and it's ilk. A lumbering statistical engine for pattern matching gorging on hundreds of terabytes of data in extrapolating the most likely conversational response or most probable answer to a scientific question. On the contrary, the human mind is a surprisingly efficient and even elegant system that operates with small amounts of information. It seeks not to infer brute correlations among data points, but to create explanations. And I think it is in this creation, uh, in this sense making, in this creation of, of explanations that, that it, there, there is the difference, an important difference. Maybe there is some other differences also exactly how the neurons are updated, but how does one lead to the other? Do you think that, that there is a direct path between these. I would say deeper explanations and the more surface phenomena that we explain, you know, the, the, the way that we explain the world, the way that we seek to create explanations, even counterintuitive ones, not once that necessarily please others, but we, we like to, and we sometimes thrive on having differences of opinions, right? We don't want to predict what other people want us to hear. Whereas, you know, right now, when in the foreseeable future, I think these, um, AI systems that we've built are precisely that, right? They're trying to match our expectations. Yeah. So, so could you speak to that? Is there a difference in design that leads to a difference of process?
Srijit Panja:Yeah. Uh, yeah. So I think, um, it's, it's well formulated. Uh, the only thing is, uh, um, from calling them, I mean from, from looking them as. Looking at them as statistical machines. I think, uh, with the advent of more language models and models in any space, it has moved beyond that a little. But, uh, it's not just plain statistics, but yeah, to still, to some extent, it takes help of, uh, um, large purpose of data that is available online, uh, that is ordered, that is open source or somehow retrieved and everything, uh, it, it takes help of that and from that tries to generate. From that, what, what is, what the, the credit goes in those parts where it is even from understanding from large sets, large collections of text or these things, it is being able to generate its own answers, right? And how that is happening is, is, is not exactly how brain does that, that is where the fundamental difference is. The generation in this case is happening by, by means of that. That's a mathematical way of doing that. It's simply, uh, to, to explain it quickly. So suppose, suppose we have a distribution of distribution of points, right? We, we have a distribution of data points and, um, so suppose, uh, uh, one of, uh, minus three point minus three, minus three, and a 2D space, minus three, minus three, minus three, minus two. This way, we have all the points, right? So if, if you try to sample out some data out of, uh, these distribution of data points that is very interior to the distribution itself, which is not very much away from the distribution, that would suggest that it would have the same property property as, as these points. But it was not the actual data point. It was not the actual points one which it was trained, it is generated, it is synthesized. So that that is how these language models actually be work on the genetic space, be it, uh, uh, not just language models and, uh, I shouldn't use that term, this generative models to be synthesized, be it in code quarters. Most of most of them are encoder decoder models with traditional and quarter or, or traditional auto andt, these models, whereas brain generates that, uh, generates that by, by, uh, uh, how, how to say that, I mean, By connecting events like suppose, suppose I, I'm seeing something, suppose I'm seeing red, okay, suppose I'm seeing red when, whenever I'm going by road, I'm seeing the signal that is red. And for the first time I saw red, I, I, I saw that the cars had stopped. All the cars stopped. All the cars stopped. Next time, again, when I'm seeing red, I know all the cars stopped. So these are two events I'm seeing The cars stop, I'm seeing the, the red happening on the, on the flashing, on the, uh, screen. So these are two events. So according to Al, also, uh, we should like, uh, use this thing here. So according to the hebbian theory, basically neurons that fire together wired together, right? So these, these two, these two, uh, neurons keep on firing at the same time, keep on firing at the same time. So now whenever I see red, suppose in somewhere else, I might be reminded of that. Right. I might be reminded that, uh, uh, uh, car should stop. But again, there is some more reference to that might, might not be exactly on the screen. So there, there are some properties that are, uh, not exactly, uh, uh, how, how it was displayed on the, uh, traffic signal right this time. So might be somewhere else. I see that. So that way it, it connects between two events, connect between three events, connect with multiple events at the end, and it, it picks up which one to take based on, uh, suppose, suppose, suppose I, I see again, um, and in another traffic signal I see the red glowing and, uh, I, I, this time know the car should stop, right? But when I, when I see, uh, red in, in someone's shirt or someone. That, that traffic, that that, uh, that, uh, car stopping, that that neural pathway wouldn't fire that much this time. Might fire a little, wouldn't fire that much. So based on that, this, this, uh, measurements of how much this neural pathway is firing, this neural pathway is firing there. The, the generation happens that way. I might take up this, like I, I might take up, uh, I might, uh, uh, I mean from, from this new pathway, I might take up some information. I might add this there, but when I see in someone's shirt, I, I might not say that the card should stop this.
Johannes Castner:Mm-hmm. Well, you also have an inner dialogue, right? You, you tell yourself. So, I mean, my experience of this is, anyway, I see something and then I create a theory from it, right? I create a theory. This is, I think what Chomsky is referring to. This is I see a red light, and it's not that I'm just seeing this statistical in this particular area. Of my eyes. I see the red light. And I know this, this must be because of the cars. The cars stop. That's what a neural network would do. Exactly. But my mind
Srijit Panja:would not do that. Exactly. I mean that, that, yeah. I, I mean that is why you, you'd see, uh, arts, humanities, metaphors similar. Such relations. It's very natural to humans. Yeah, that's right. So even, even two things that are not dis not very much connected support a death, suppose a death shirt or, uh, in this example itself, car stopping. It's not really related, but maybe by, in, in a, in a poem, I might. Connect them together. Yes, yes, yes. While, while, while, while Judge GT or these kinds of models might have not seen that in, in the, in the, in the large corpus of text and sampling them out based on distribution, which would be top in that way.
Johannes Castner:Yeah. Yeah, exactly. While they're also never felt or saw the would be color red, the way that we see it. Right. So, so this is another thing, and that, that relates to consciousness. We have, we have, we are different from them in the sense that we have consciousness and they do not, even if they pretend to have it, they have not felt the wind in their hair or the have not seen the color red. In reality, they might have seen some frequencies or some numbers that were related to them via some input sources, but they, they don't have an experience. And I think that this relates to this as well. Right. So if you were to know exactly how the brain does it, Do you think we would have consciousness or do you think that consciousness itself is a, uh, so, so I, I see that there are two schools of thought here. One is that the brain does produce consciousness, and one is that, uh, consciousness is a, is a fundamental, uh, property of the universe that is somehow channeled by the brain. So, uh, these, these are, these are different views. Um, but it is interesting to note that there is no difference between, as far as we can tell with our instruments. We cannot measure any difference between the co conscious and the unconscious brain. And that's quite, um, quite something. So, so do you think that, um, that this is significant at all, this consciousness in, do you think that this is something we should worry about and that's why we should build more brain-like, uh, Architectures rather than just brain inspired arch uh, architectures. Do you think we should build conscious machines?
Srijit Panja:Yeah, I mean, uh, yeah, definitely. That, that is, that is of a very prime question. Uh, so how, how, how I see it is like that these schools of thoughts, like, uh, right, uh, uh, one where, uh, consciousness is is a product from brain and where consciousness is something that is in non nature. These, these, uh, schools of thought, I, I think the one, uh, it, it basically stems down to, uh, it's exactly like viewing, uh, brain itself from a neuro scientific point of view during neuroscience and from a psychological point of view. So, uh, I, I totally go with, with the scientific part of it, right? So, uh, uh, I, I, I prefer to see that whatever we are seeing, uh, whatever we are experiencing, whatever we are remembering, whatever is triggering next time. So this is, that, this makes me conscious. We can put a term to it. We can say it cons, that we are conscious that way because we are being able to, we, we are being able to understand the past, present as well as the future. Uh, so we, we are there, but there's a science to it. There is science in the way that it's, it's a neurons that does that. So for every, for every, uh, event that happens, right? However I experience it. However, I take that as an input, be it using, I speed, using ears or any of the five sensors that maps to a neural pathway. There's an encoding, uh, there's encoding happening with, with the, uh, electrical impulses. And so for, for each kind of event, there, there, there is this mapping inside my brain and, uh, uh, there's some neural pathways for that. So the, the question is, are there neural pathways? Uh, I mean, um, by birth? No. That neural pathways keep on developing, right? If, if you do not see an event, or if you do not see a person for a very long time, that neural pathway will gradually degenerate. Also, the signups will, uh, be removed, will, uh, will gradually diminish and annual neural path will go off. So consciousness to say, uh, that it is from, by or from nature, that that would, would, wouldn't be, I mean, a, a, a very, uh, very, very correct way of saying very, very correct. Uh, I mean statement to be, be very precise. Uh, it, it's. Evolutionary, it's developmental. Um, it's based on how, what we see, what we have seen in the past, and how we relate to them. And it's all, all, all the, I mean all, all, all credit to the, to the
Johannes Castner:neurons, but there is this difference between intelligence and and consciousness, right? So you could have in theory, I mean not just in theory, but also in practice. You could have someone who's highly conscious of, of, or not of themselves and, and of their environment, but at the same time cannot calculate anything, say anything, even speak the language, do anything behaviorally that is very interesting, but be extremely conscious. And on the same time, you could have someone who is, is, um, very intelligent. Like ChatGPT is rather intelligent, right? It, it sort of seems intelligent. It could probably pass some IQ test. I mean, at least the newer versions, GPT-4 or maybe GPT-5 will be able to do it. But at the same time, they don't have an inner story, right? They, they don't have an experience. So, so I think that this difference between intelligence and experience is one that's really important. And so, um, because I think also for ethics, right? So when we, when we decide, even for abortions, the discussion often is, but when does it feel pain? Right? When does it start feeling pain? When is it conscious? When does it start feeling things and be, and feel as a self in a way, as a, as an entity distinguishes itself from the, from the environment? When does that happen? Right? And not, not when they should start playing piano or when can she beat me at chess? Those are totally different questions. They're on a different, a axis as I see it, right? So the intelligence and consciousness seem to be not. Necessarily that related. You gotta, you see like in religious experiences, a strong could be like a strong conscious experience, but it might not be all that intelligent sometimes. So, um,
Srijit Panja:yeah, I mean, I mean on, on, on that front, what I, what I have to say is, uh, I mean, and, and, and I mean intelligence is, is something how, how, how I have tried to like put it, or also, I mean, uh, war basically is, uh, the ability simply to, um, to, to detect and predict, so a person we say is intelligent, uh, or, uh, by that matter in any, any living feature is safe to be intelligent. If it can see something, uh, that it is, it is there, uh, by the, by ordinance and everything. And then it can predict what should I do with that next? And that comes from the training. Like suppose I have, I have, uh, used laptop, uh, for several years, and I see the key. Now I know that I need to test this. If I have not used this, I can detect, but I will not be able to predict. So, uh, that is how it, it relates to consciousness. Um, I, uh, so we, I I'm in the state. I, I'm, I'm conscious of the fact that the, there's a laptop and then I, I'm being able to FedEx that, uh, FedEx what should I do with this? So again, uh, I mean, to, to be very, to be, to be, uh, to, to put it correctly, it, it's, I mean, nothing would be there basically if, if we hadn't been able to, uh, I mean, uh, perceive that in our thing. They,
Johannes Castner:I don't know, you have a chess playing algorithm, right? You can have a chess playing algorithm that understands exactly what to do with all of the pieces on the chess board to beat you, but it has no experience at all and even has no real reason to play chess at all.
Srijit Panja:Yeah, yeah. So, so that, that, that, that is very much programmed. I mean, that, that, that is very much, so suppose there is this rule and it sees that, okay, next time when this is the condition, I shouldn't do this, I shouldn't. So it, it keeps on training itself, but what the point is, it, it would not be able to, uh, like do a lot of things at, at the same thing. And that, that is the scene. Also, we, we see that, uh, that, that's like the example I said. So, uh, two, two things. Fire. So two, two things fire at the same time and we are being able to delay that. So multifunctional roles and being an overall kind of a, an overall mechanism. That, that is very, uh, that that is very innate to how human intelligence works, or that's for that matter, most advanced animal
Johannes Castner:intelligence. Oh, but you have self-driving cars, right? So for example, that can drive better than I can, can drive better than the best Dr. Human drivers can drive already. And so, or maybe not exactly already, but. In 10 minutes from now. Right. And, and so, you know, how do you explain that? Right. So to me, and, and that car could have in that ChatGPT, you know, built into it. And then it can also talk to you now and then you have, you know, text to voice and then it can also speak to you directly with the voice. And now the human imitation gets better and better. So I don't know that this behavioral answer captures it all. So what I'm saying is that even if I'm behaving exactly like myself, if you see something that exactly behaves like me, and you predict everything I do, and it will do exactly the same things, but it isn't actually conscious. It has no inner story, it has no inner experiences and it cannot feel the wind in its space. I think there's a big difference there in this thing, you know, and, and that is not explainable by behavior. So that's what I think is the interesting part here, is that, that there is something very important. It's what ethics is built on. You know, if it's, if it's not conscious, you can blow it up. It's not a crime if it's yours, if, if it's not yours, it's property damage. But, but, um, it's not you. You see, it's not, it's not a murder. Murder. It becomes murder if it's conscious, I think. And that's, and so that, because that's very interesting here, because I think if you, if you're building brain like architectures, you might actually build something conscious, right? That's, I mean, that's a question. I mean, I, I don't know. Do you think that's true? Do you think that it will develop its own inner stories and feel the wind in its face?
Srijit Panja:Yeah. Uh, and I mean, from the point you started, right? Um, uh, self driving car. So that, that brings me to a very important point. So, you know, you know why I mean, and most of these. Unique purpose. Uh, like there is one, uh, I mean, uh, not mon not multifunctional roles. So self-driving cars drive very well, right? Yeah. So, so that, that is a boon actually. So why is that? Because compared to, we have only 1, 1 1 brain here. Right? And so based on that, suppose I'm, I'm driving something and I am seeing suppose a phone comes or something come, the same brain is again taking that also. Yes. Right. So while, while my driving for that two single pathways were being already hiked or, or they, they were already functional. This thing, the phone ring also the new pathway that is there for that phone ring that also now hikes, right. So the overall napping now changes and it's not remaining just the, the, the brain or, or the model or, or the neural pathway for driving. Whereas in, in the other case, it's, I mean, in, in self-driving cars, it, it don't get the stuff. Yeah. But I
Johannes Castner:don't see the significance actually very important. I don't see the significance of it. Because if you can behaviorally produce the same kind of phenomenon like a human being, you can build a robot, right? And you can put into it a module that drives a module that speaks perfectly well. Reasons perfectly well can do math, can solve proofs. You can put all these modules in there and it'll behave much more intelligently than any one of us because it can solve any one of
Srijit Panja:the problems. But then again, you cannot. Yeah. But then again, you cannot relate in between two. That is the, that I'm talking about. You cannot relate. So suppose
Johannes Castner:you, you. What do
Srijit Panja:you mean? Yeah, so suppose the, the, yeah. Suppose there is a, there is a part that talks are, uh, is, is, uh, related to speech and there is a part that is related to, uh, seeing, right, uh, vision. So now when, how, how does it work in brain? These are not separate. So when, when I am seeing something and the person is talking, the person I'm seeing a person, the person is talking. So I'm seeing that person with my eye. And immediately at, at that instant, I'm also hearing that the person's voice with my ears. So both the neurons, both the neural pathways are firing at the same time. So that would not be the case if I create two
Johannes Castner:different ones. No, but you can easily, right now you can have a YouTube, uh, um, Let's say add-on or some kind of, uh, you know, video intelligence that can tell you, oh, there's Johanna, right there. He is talking. He, and, and he can do it all in real, real time already. You can even say, oh, now he's smiling a little bit. He's got, so, so you have all of that already in the way, you have that already. Right. And then you can even translate it into Italian if at the same time, in real time almost.
Srijit Panja:Yeah. But you, you again know that the data for that, uh, it's, it's generative, like how we just explain how we just, uh, caught upon it. So it, it would still be that, that kind of a, I mean, uh, that kind of a distribution based generation. Yeah.
Johannes Castner:So the mechanism will be different, but observable, what we observe will be the same. Right. So it can even outperform us in, in terms of observable performance in almost, I mean, in many tasks it can, well, it beats the best chess player and in, in can, you know, beat the, you know, so, so it can outperformance in some, some dimensions. Sure.
Srijit Panja:In, in Egypt tasks, in one purpose task, then, uh, in, in most cases,
Johannes Castner:yeah. But what, what I'm saying is if we are building some, what I'm saying is if you stick them together, You know, like from the observation, you will not observe that there are five different modules working at the same time, and the exchange of information is extremely fast between them. So it's not observably different. Right. So that's, that's my my point is basically when you look at the thing, if you can mimic a body, if you can build something like a human body, uh, you know about, we will be there at some point. You stick all of these modules in there and it will behave like a very smart human being. You agree? And, and so that's what I'm saying. So what is the significance other than consciousness? Aside from consciousness, which is, you know, I think very important, but the significance of, aside from that, in terms of behavior, I don't think there is any in terms of thinking, you know, I mean, yes, it took a lot more data to train that thing than it trains to takes to train me because I see two cats and one dog, and I know, oh, this is a cat and this is a dog. This machine has to look at, I don't know, thousands and thousands of videos, but in the end result, once you train it all up and you look at the behavior of the machine, can you differentiate between it and the human? I don't think so.
Srijit Panja:Now what, what, what, uh, you, you just spoke about like if we try attach all those module together, uh, it would be smart as, uh, as smart as humans. That is, that that is true. So if, if we, if we try to attach all, all those models together, what we are essentially doing is we are trying to put the neural pathways together. It's as it's if you are into that, right? So if we are trying, if we are trying to create a computational model that is, uh, same as the brain, so definitely, uh, at some point it would be as smart as humans, so if, if we attach it in this way, that, uh, once this, this neural pathway fires, this neural pathway should also fire. And, uh, both the neural pathways can file at the same time, can take input at the same time, uh, that is doable and that that is what the objective is for many. Yeah. But you see,
Johannes Castner:there's still something very different about it, right? I mean, even though observably you get the same results, but what, what Nom Chomsky is saying about the lumbering statistical machine that is trying to please you. So the one thing you know, you will find is that it will please some average person or someone who's trained them, it'll have an opinion even. But the problem is that, that this is not the way that we work. Even with, even if you fire them at the same time, I, I will argue this is not how we work. We, we, we, like, like Nom Chomsky says, we, we make sense of the world. We try to explain it. This is how we learn when we manipulate things in our, in our head in this way. And so, and we don't look at thousands of thousand, thousands of kangaroos and then no, oh, this is a kangaroo and this is a cow. We don't need thousands of them. So we have a different machinery. But observably, I think observationally, you can ex, you can, you can, and this I think is frightening in fact, because I think what you're building is a zombie, something with no consciousness. Uh uh, but, but, um, but observationally, when you look at the two entities, they can actually behave frighteningly alike and maybe one better than the other. And the one that has no consciousness being the one that's better. So what do you think of this is, is don't you think that one, the, the main reason to rebuild the brain or to build something much more brain-like, uh, is, and, and I don't think it's re it's, it's even reducible to the firing of neurons. I think there are other things going on in the brain, chemically, electrically, aside from what we are modeling there, that produces consciousness that has nothing to do with computation.
Srijit Panja:Okay. Uh, no. I mean, uh, what, what essentially happens in the brain, if you even do electrically or chemically, it's the results is the firing of the neuron. So if, if there is an electrical exchange, uh, I mean from one neuron to the other, that that is basically the I understand that. Yes. What age that. What, what aids that is basically the chemical transactions in between thes coming in, coming out. Um, how at, at what input, how many iron should come or what should be the, uh, potential there? Uh, uh, with respect potential inside the cells with respect to the outside of the cell, these things is the chemical transactions. And with respect to that, this is, uh, the, the electrical inferences go from one neuron to the other, and that is how the, the, the neaural pathways are built one neuron to the second, third this way. So how, how, again, we like, um, I mean, how, how, how are we saying that we are conscious? We, we say we are conscious because, um, because we, we are being able to, uh, relate past with present with the future. Right is, is, is that a sentence you then I can proceed? No,
Johannes Castner:I, I wouldn't say it's next. I think that that neural networks and even, even, I think that even ChatGPT could do that already. It could lay out a history of, you know, you could ask it about a history of Europe and it will tell you all the different time periods. And so, but I think it is, the difference is really in this experience in the qualia and, and, and, and not just the qualia, I mean mean that maybe that's even, I think that's, that's even not right. There's this conscious field. I think this is how it has been described. The conscious field is that you relate to, um, people break it down, the ego and the it and so on. There, there are breakdowns and psychological breakdowns of it. But the, the, the, the fact that you experienced your environment and you realize that you are, you know, you are, you are Srijit and not someone else. You know, you're not me, you're, you're, you're you. Right? And, and you, um, and, and that, that identity. It's, you know, the, this fact that you're experiencing the world, right? I think that makes all the difference and it's, I don't know that it's a computation at all, to be honest. I think that the computation, so, so the way, the way that, um, uh, Utpal thought of it is, is basically that the mind is feeding. Consciousness, all of its experiences and all of that is computational. So essentially the there is a subject inside of you or you know, whatever. However it is, however it gets there. That's the interesting part. I don't know how it gets there, but we are, we are subjects, we are not objects, right? We're, we're, we are thinking, but we are, we are, we are, we're not just thinking we are conscious. So this is, I think this is the, the, the ultimate difference that Descartes even had wrong, right? The, the, the fact that what, what makes us ethical objects, you know, what makes us the object of ethical discussions of what, what is the good thing and what's the bad thing to do and so on. All of that re requires consciousness. So it, it is about conscious objects, conscious objects we call subjects. So we call them subjects. They have subjective experiences and because of that, they're significant, they're ethically significant, otherwise they would not be. And this consciousness happens even with people who are very still and do not ob, where you cannot observe any behavior from them at all when they meditate. In fact, it is said that the consciousness is heightened, whatever that means, exactly what that means. It's not clear to me. But, um, you certainly have different experiences and there are some things that are going on in the brain when you meditate and all of that. And those are computational processes and I agree with that. But the subject that is in there, I don't know that it's a computational process. I think there is something else going on either in the brain and it might not even be a process. It might be a still thing, a thing that's rather still that, um, that, that produces this effect, if you will, that everything else depends on.
Srijit Panja:Uh, I mean, I, I don't, I do not see it from a divine point of view. No,
Johannes Castner:sensitive. I'm not saying that either. We can study it scientifically, but what I don't agree exactly is that it's computation. So I think there's another process or a thing in, in there somewhere. Maybe not only in the brain, it could also be partially in, you know, whatever the whole body. In fact, uh, you know, we know that neurons are firing all over the body, including the gut, uh, in particular. But, but, so I'm not sure where this thing is located or if there is a location of it at all, but it is some process or some organ. Some part of the brain organ likely is what John Searl, attributes it to the brain. He just says something in the brain that produces it. But he doesn't necessarily know that it's always the firing of the, of the neurons, which I think is, is responsible for intelligence. The firing of the neurons is probably responsible for intelligence.
Srijit Panja:It's, it's not actually, it's not actually always the firing. Uh, what, so what the point is that each, each time, uh, the neural, neural pathways gets formed is so for, for Korean neural pathway need, we need a series of neuros, right? So there we need the firing from one neuron to the other, right? And from next neuron to the, but even if the neurons don't fire, even if the neurons don't, suppose the neuron is not firing, but still, I'm, I'm giving some input and there is some rise in, in the, in the, uh, what it's called, the, the potential there in inside the, inside the cell. So maybe that is not crossing the threshold, but there is a spike there. And that is also part of the mapping for this, this particular thing that, that, that was the input. Uh, so for it, it, there is no need of that kind of firing, but yes, the, the neurons should have this, this potential, this changes in potential. And that is how for, for each kind of an object outside for, for each kind of an experience outside there is a unique, um, uh, embedding or how we call it encoding, uh, uh, or mapping in, in, in, in the brain as well, in, in, in, in combinations of neurons. So what, what, what is what? Yeah. Or what, what, what I want to say is very, I mean, very much is what is very fascinating is, uh, I mean, whatever we see right now, right? Uh, suppose, uh, uh, we, we build, uh, building or we, we build an office building. We, we have cut, uh, created a park or anything. Some person before doing that, thinks about that, right? That, this way I will build it. So e even before building it, there's a data that, that is inside and. We, we create that, we recreate that. So these are some experiences that we have taken from outside. And based on that data, we are again, creating new data, uniting new data inside our brain. And we are again, recreating
Johannes Castner:that in. But, but this is not entirely true. Right? We noticed also from Nom Chomsky, so even in the beginning of our life, before we even have any experiences at all, we must have some, uh, some potential for language learning, which is very unique. So, so, and this, and this is different from chat, G B T, uh, and this kind of tools because, and this is what is argument is these guys would also work on languages that are impossible for humans. So there's a, there's a range of things that are possible for humans, and it is based on something in the brain, for sure, something that develops in the brain, something that evolved in, in the brain, but it is pre-coded. It's hardcoded, it's already there. So I think that a lot of things that make us who we are, the shortcuts that we, uh, that we can take are hard coded even before. Uh, so, so this is the, the, the, the, the, the, the nature nurture debate. You know, and I've, I've read a lot of books on this and you know, that, you know, Stephen Pinker even agrees with me on this one where he doesn't, he probably wouldn't agree with me on the consciousness one, which is, I think they have a, a little bit of a funny, um, uh, idea there. Uh, but, but, uh, in terms of the, the, the, the fact that we have some, that we have a lot of innate abilities that we don't fully understand yet, we are much more efficient computers. Than, uh, than those machines that we're building. That's also part of it. Right? So as you're saying, it's, it's neural pathways. It's definitely that, that is all true for, for what I think is the mind, you know, the machinery that makes us pretty efficient at learning, driving and all of these things where these machines have to learn this. They have to drive thousands and thousands of miles, either in simulation or in reality before they can drive anything at all. And we can do it pretty quickly. And all of this has to do with a combination of what you said, that this neuro pathway learning mechanism is better, it's more efficient. And then also because there is already so much architecture built in to begin with, all of our drives, you know, all of our, the, the drive to survive, the drive to have children, all of these kind of instincts that we have, right? They're also built in AI will not have that. So it's, uh, it's, it's kind of.
Srijit Panja:These are basically, yeah, I mean these are basically part of, uh, how, how the human body is that, is that would be this, that we can, we can't deny that. No way. So, uh, I mean the, the hormone exists and so all the, all the drives that, that would basically, I mean, how, how the human body works mm-hmm. But all, all the development of things on the other hand that, that we pick up on the process, uh, from experiences, these, these creates, these pathways that, that we are talking about the, the learning part of it. Mm-hmm.
Johannes Castner:That is true. Yeah. So, so you are, you are focusing on the learning mechanism, and I think that's very good. And I think you're right that we can become more efficient liquid neural networks. Have I asked you about those yet? They have been inspired by bio biological brains, I don't know the name of, of this worm, but it's a little worm. And it's very much built as a copy of it, a digital copy of it, essentially. And it has fascinating properties and then it can rewire its architecture relatively quickly and learn new things rather quickly compared to previous neural networks. So there is some, there's some work there. Are you aware of this and what do you think of this work?
Srijit Panja:Yeah, I mean, uh, the, the, I mean, when, when we talk about these, any, any kind of such networks that are, I mean, biologically very much secured. So we, we, we, we start from, the first thing that started with, uh, we, we started with this field was the spiking neural networks. Great. So, I mean, uh, uh, the, the spiking neural networks, uh, we, we got. In conventional deep neural networks, the, the weights are very discreet, right? Uh, whereas just, just as we talked about right now in brains might not be firing, but still, it, it, there is a little spike. And when there is a removal of that input, the spike drops and there is a removal of, I mean, uh, again, again, that push of that input. And there is again, that spike and also the intensity of the input matters, not just how many times or how many it is. If it's high intense, maybe just one time might give a very high spike. So based on that, how spiking neural network was work very much, very much close to, uh, the, the veins. So, I mean, I, I, I, I've seen this work actually, like why, why this is something that I very much enjoy discussing and talking about is I've seen this kind of a, I mean, this kind of an implementation in the hardware while I doing my masters, right? Um, and, and so, um, and in the lab where I was doing masters professor there and everyone, the, the team was very, uh, uh, we, we had a hardware lab. We are trying to put, uh, these things inside the, the, the idea of spiking neural networks inside mes. So if I, I don't know, like, uh, so there's a certain, uh, uh, fascination about, I mean, is certain a good thing about members? So, uh, ministers are, are, if, if you know about this, um, it's, it's not very much, uh, popular as compared to registers. But the, the why, why this thing, uh, is, is very much. Uh, I mean, uh, uh, relevant in, in the world of creating the, the brain-like structures is basically it, it holds that resistance, right? So, um, suppo, suppose you pass a current or something, you, you pass a current or something, you, there's a resistance, which is very much fixed to, uh, which is very much static to or to the property, to, to, to that particular component, to that particular material or the weight or that thing. Whereas members stairs, uh, it, it keeps on, it depends on the current as well. So if, if the current, this time is increasing, so it, it, it, it, uh, keeps so, uh, it, it keeps how much the resistance was previous time based on that. When the current I pass next time, it helps the current to pass next time. Right? So there, there, there is a chaining in inside this hardware, which, which is very much interesting. Um, so that, that is what we, we saw, uh, we, we worked on this districts and those things. And, uh, I mean, uh, so I, I have seen several people, like, it's, it's my very good fortune I'd say that I, I've been able to work with, I've seen some very good people working the areas of AGI to be, be, get precise, um, the master. And, and after that, also currently, if you've heard of this lab called, called Numenta, uh, so, uh, it's, it's in, um, it, it was I think previously called, uh, Redwood, uh, center for Neuroscience or lab for neuroscience previously. So, uh, um, the, the inventor of Palm Top, uh, Jeff Hawkins. So he, he was initially, uh, uh, built a palm top and then he was very focused in neuroscience. He, it doesn't have a formal degree in neuroscience, which was very fascinating how when he, when uh, we get to hear from him, How, uh, I mean, um, what, what kind of computational model should be there, with respect to brains. So he talks about a very, which is not something that, that I have been talking about right now. It includes a natural level as well. What are the layers of the brains, right? So it, it's called hierarchal temporal memory htm. Right? So that, that is something, uh, where, where, exactly. So what we have been talking about so far is on a neuron level. So how, how neurons that learning, uh, electrical impulse neuron helps in learning. So it is also important that how we place these neurons inside the brains. What are the locations, which part of the brain is for which thing? Which part is for the other thing? So that is the kind of thing that is, that is a, very important, uh, concept in htm, uh, hierarchical temporal memory, so, uh, I, I, I follow that lab very, very, I meanly, uh, whenever there is a newsletter or something. So yeah, very few people working on this, but I, I'm sure they are very, uh, very big supported of AGI and yeah, brain accurate components
Johannes Castner:What you think of this, so John Searl makes this argument, right? So if we simulate fire, we are never afraid. When you look at the computer and you look at a simulation of fire that it's gonna get hot or that, you know, if you simulate explosions, you're not going to step away from your computer every time you press the button, right? There is a causal effect that is missing from a simulation to a, in, in a, uh, from that sort of simulation. In our world or in our simulation. Some people think we are in a simulation, but maybe we are. But if we are, then we are on a higher level of it, that when we simulate something in our computers, we're not reproducing them. Right? So if we, if we want to fly from London to New York, we will go to a plane, right? We're not simulated on our computer and hopefully we're gonna get to New York that way. So in that way, simulation, do you think simulation is sufficient to really reproduce something like the brain? Or do we need to actually build physical things? Because, so also even the way that the brain works, when we are then starting to look at the chemicals, neurons don't just fire, are not fired. They're not just zero, like you said, they're not, they're also not just continuous or spiky, but there's something else to it. They have multiple, um, multiple chemicals that are not just one chemical but multiple chemicals that can affect it. And in fact, you know, if you take certain drugs or certain stimulants or certain. Uh, chemicals, they will have a direct effect on your brain and you will have very different type of consciousness, in fact. So how do you, do you, do you think that we are working at all in this direction, or is it just simply the one-dimensional? Because I think at the moment this is still very one-dimensional, right? It's, uh, firing a little stronger, a little less stronger mix and imprint makes no imprint, but it doesn't, or makes a stronger imprint. Maybe a degree question, but not one of three or four different chemicals having different effects at different times. What, what do you say to that?
Srijit Panja:Yeah. So, uh, there are two questions here. One is, uh, simulations, right? Well, well are simulations enough? That is a very, very, very valid, very, very valid question. So, uh, what, like, uh, I, I think it, it depends on the infrastructure. So, uh, I, I mean, yeah, so it, it, it depends on, and, and how many dimensions are we being able to, uh, uh, able to simulate? So suppose, uh, yeah, as you said, like there's an explosion or something, so we do not get, uh, frightened or, uh, we do not. So it, it's basically because it, it also depends on how we feel it on our skin, how, uh, how it, uh, how it sounds, maybe sound, uh, uh, sound can be done in simulation, but all, all the features are, uh, not at, at this, at, at this scenario involved in, in, in the simulation. All factory sensors, um, uh, this, uh, touch sensors, these kinds of sensors still, still needs to be evolved to our, to, to very large degrees. Uh, which, which is not the case right now. So currently we, we, that, that, that is a trade off that we, we, we face also in our day in, in, in the kind of development works that we do. But going forward, I mean, um, I I, I've seen some good, uh, developments in the all factor, uh, uh, I mean census. So, um, there is computer vision right now. There's national language processing right now. Uh, so, uh, computer vision, it's, it's basically, uh, how, how we see it so sound, uh, um, so, uh, speech processing, these things, right? So when, when we can also, uh, make, uh, smell, uh, also a valid. Uh, feature for computers to be able to perceive that that would be one more step ahead in, in the simulation. So I I, but
Johannes Castner:even if we had that, we still couldn't go to New York based on that, right? So we, we, we would still go to the airport, to the real airport, going to a real plane and really fly to New York. It's not possible in the simulation, right? So, so this is what, you know what John Searl makes this comment, and he says that's the difference really between what we're doing when we're simulating the brain. When we are really, what we really need to do is we need to reproduce the causal powers of the brain, and only then will we have consciousness.
Srijit Panja:So he, here comes the question of, uh, the, the, the very, um, uh, very question of metaverse, right? So e even even sitting here, can I see London or can I see, uh, Antarctica, any such thing? So currently, no. Uh, can, can we experience Antarctica as it is, uh, as good as if we had gone there, uh, by sitting here, surfing Metaverse? No, because we, we cannot simulate to, to, to, to include all the features, all the, all the five kinds of features to, to exact to that level. But if we can do that, then, then there is a, there is very much hope in, in, in that, uh, direction. Um,
Johannes Castner:but, but that still would be different, right? Because I mean, you're saying that if I, if I wanted to be in San Francisco, I could simply. Go put on some helmet and experience San Francisco, but I can't just go to a meeting in San Francisco and knock on someone's door and say, here I am. Uh, you know, that's, that's still a difference, right? I mean, it's, there is a real place called San Francisco
Srijit Panja:that, that is, that is geography. So what, what what we can at most to do is maybe we cannot, uh, I mean, um, what, what we can at most do is, is to try to create the perception of it. Try to create the inner mapping of it.
Johannes Castner:You see, and this is, I think the yes. And the simulation, right? And so then what if you do this and you expect this to building a consciousness? And you can say, well, what we can do at most is to create a perception of consciousness. But, and we can do that probably quite accurately, but I think we cannot actually build the real thing unless. We build something that has the same causal powers of the brain, and I think that I'm with, with, with John Searl there, or I'm not sure because it could also be true that, uh, that consciousness is simply a, something like radio signals, right? So something different, right? Similar to that where, where the brain is simply a receptor of it and doesn't produce it at all. I think that's still a scientific, very scientific possibility that, that, um, that, you know, there are, there are such things like radio or, or you and I, we can speak here on this phone, and it's all based on things we cannot see, right? There are whatever 5G waves or whatever that gets us from, from one to, to, to a receptive power tower. And then from there it, it sends it up to, um, to some, some satellite, right? All of these things are not, if, if, if you didn't know about this. You would think that that's magic, but so similar, similar to consciousness. We don't know how it works, so we think it's a mystical, spiritual, or, you know, we, we, we look for the Vedas to answer that question. So maybe there's some something real in them actually as well. I'm, I'm not even doubting that. But to say that just because we don't understand it means it cannot be scientific. I think it's also not true, it's just that we cannot currently understand it. Right. You know, our science couldn't understand radio waves. 200 years ago we didn't understand anything and so now we don't understand consciousness.
Srijit Panja:No, no. It's, it's, it's, it's very much understandable that scientific, there's no doubt. So, uh, how, yeah, how we see it basically.
Johannes Castner:Yeah. But just to say that it's not neurons. That are doing it. It's not to say that it's not scientific, right. It can be something else. That's all I'm saying. And I think that's what, what I think the causal powers there is this difference. We, we can simulate, apparently we can simulate intelligent behavior, and that's amazing. That is something I would not have thought. I, I must admit, I would not have guessed this. Or we can do it, but consciousness, can we simulate it or do we have to build a machine? Do we have to build a particular instant? So the question really is, is it substrate free? So is it, can it be done in a way that is devoid of any material replication of a specific type?
Srijit Panja:No. Again, like, uh, uh, I, I would like to iterate on this part itself. Like, uh, what, how, how I see it still, it's, it's evolution. Um, any kind of an, any kind of, uh, action or any kind of thing that you keep on building, right? Uh, so you, you give an input, you give an input, you give an input. Next time you know that this is this or this. So how, how do you, when, when we say about consciousness, are we saying about, uh, well, we, if we are seeing it in this respect that we are conscious because I know that I exist, like I'm aware of myself that, that, that kind of a notion. Then also brain content between like, uh, so, uh, I have my experiences I like, and any person has their own experiences with different objects, different things, and there's an exist. He, he can relate to all those experiences. Uh, at the same time there, there's a, he, I mean there is he in all those stories, he or she and all. So I mean, yeah. Uh, it's, it's all, I mean, it's, it's calculated in the brain, in, in all these, uh, instances and how, how that that is already, uh, that that is spoken about, uh, so far,
Johannes Castner:but in neuroscientists don't fully understand it yet. Right. And there are two approaches in fact there. One, one being the study of, of a conscious field approach, which I, I prefer, I think is a more, um, Probably the better one. And then there's this other one that tries to understand qualia, which is this idea of individual experiences. You know, how does a human experience the color red? How does that work? That mechanism? But I think that once we understand the conscious field is, you know, how is there this I there? You know, how does this happen? This experience of the self and the experience of the world. Once we understand that we probably will understand these other things in a more, you know, pretty easily. Like once we understand, you know, how does conscious field is there, then you know how to experience the color. Red is probably gonna be trivial compared to figuring out how this whole self got in there to begin Well, do you think it's dangerous that if, like, do you think it's dangerous to build machines that are not conscious and to. In such a way that they're indistinguishable from conscious entities.
Srijit Panja:How I, I see it is it would be actually when, when we see indistinguishable, um, it would be actually indistinguishable. That is, we cannot say whether it, it is, it is a human or it is a machine is, is going to brain way me. If, if we try to compute, uh, I mean try to create a model that works, that works brain. So that in, in that respect, we can then, then we can say that exactly the exact things. So I mean, with, with that respect. So there, there is no such danger because creating humans also has, I mean, has its own trade offs. Like, um, we exist, we coexist, right? I, I, I am very, uh, I'm a very positive, um, kinda a person. So, um, like, so, uh,
Johannes Castner:well, I challenge you on this one because, I mean, on this indistinguishable one, this is touring's hypothesis, right? So to touring, put forth this idea that we could test. Whether some, whether a machine is conscious by asking them certain questions and by, you know, once we, once we feel that they're human, once we, once we detect them to be human, they must be like that. They must be conscious. But it turns out that, that this test has been passed by machines that I am very sure are not conscious. So, so, um, chatGPT has passed basically, well, the, the GPT-4 for sure has passed this, this test and others, I think this Google, there was a Googler who was fired because he said that the, um, that the, that the chat bot was sentient. But I am very sure that it is not sentient, but it behaves linguistically the way we speak. It behaves as if it was conscious, right? And it is impossible. And it becomes even more impossible over time as they get better to test, to tell the difference by testing it, by interacting with it, by poking it, by asking it interesting questions or even questions of the theory of mind and so on. They will pass all of that. Because it is, you know, because that's how humans talk when they talk, and it has so many texts. To refer to. It can simply produce the statistical patterns that a conscious human being would produce in, in response to ask, uh, questions and so on. And very likely we will be able to do the same thing with little face switches, little smiles. We're already quite there as there's a frightening video of, of, of such a, um, um, virtual, uh, human who has passed the touring test and all the derivatives of it through all the different theories that might be used to test consciousness all past it, and it yet is not conscious. So I, I am there. That is the thing that I'm frightened about because I think that if you fall in love with a statistical machine and you don't know it, I think that is very, that's ethically, completely in that's. Go really wrong. We should never be misled to, to live lives with meaning, try to create meaningful relationships with things that have no meaning, that have no internal semantics, you know, that don't understand the world as an experienced world, but only one of a statistical correlations kind of world. And I think it's amazing that we can do it. It's frightening. It's definitely disproves some of my hypotheses, but I'm still don't think, what, what do you think of that when I pose it to you this way?
Srijit Panja:Yeah. So no, I, I do not see any kind of, uh, I mean, when, when suppose uh, we, we, we, we, we are creating something, right? We are creating something, uh, very much, uh, for one purpose, as, as I'm saying so again and again. So, uh, so. We are creating something for one particular purpose. It, it is designed not to get distracted. It is designed not to get, uh, other, other neural parts or however, its intelligence is, it's mode of intelligence is to, to, it's, it's not, it's designed not to include other data at that time and not to act with, with respect to the data.
Johannes Castner:Hold on, because I, I can read to you what it says here on open ai, what it says about it, and they are saying the opposite. They're saying in fact that they want as many use cases as possible to be tried out on the same algorithm and that they're now, the GPT-4 is basically their, their attempt to get closer to agi and, and they want to use the same model to solve. A lot of different problems, like for example, manage a company, it it might be able to manage a company. In fact, in China, there is a company now that has made an algorithm their CEO and it's beating the stock markets. So this is, this is actually not quite the way that you're saying because...
Srijit Panja:let, let me hear this. So, uh, what, what, what do, what do we mean by use cases in, in that case is, uh, so they, they have, I mean, there's a language model or not, not, not this language model. It's a pipeline basically. It's a, uh, such that it's conversational generated, uh, contextual. So based, based on that, we can create a, a number of things that, that, uh, has the same inner working and based on, on top of that, we can create a number of things. Like for instance, when there was this language model board or these things, We, we, there, there is always this option to create either a question answering, model question answering kind of a pipeline. Sentiment analysis is kinda pipeline. So even on one particular thing, we can create different use cases. They're also aiming that because currently ChatGPT or GPT-4 or, or the, uh, generative pre-train transform models have, have, I mean they, they, they, they have been on the site, right? They, they have been on, on their site and, um, not been used too much in, in, in, by the general public or the corporations or anything. So with the advent of this ChatGPT and with the popularity that it has gained, and it's really fascinating to see the kind of intelligence, whatever be the process had been followed, but it's still a kind of, I intelligence be a statistical, not, it's not statistical there, it's more than that, but whatever kind of intelligence it is. Uh, so it's, it's good to see that. And based on that, we are more, uh, open to using that. They're also more open to let us use that. Uh, so, uh, so on that context, there will be a lot of use cases of, of this, uh, GPT models. Yes,
Johannes Castner:yes, yes. So, I mean, I think in the dis indistinguishability between the human and them, it gets, it gets tough. I mean, you know, if you can do your accounting better than your accountant can do it than, you know, what is that, that is a replacement directly of a particular job, of a particular human being, that it's, it can, you know, it can answer emails, it can do all of those things. Could you le leave, I would love it if you could say something to the audience, you know, something you would leave them an impression with. Um, it was a little bit of a debate, it was interesting. Um, it would be great if you could. Yeah, I mean, uh,
Srijit Panja:to, to be, to be very honest, I love debates more than conversations. So we, we, we get to see the best, best size of, uh, like both the, both the people in dialogue. Or else to be, to be true. Like, um, it's, it's, uh, it's, it's like someone is ready with some questions and he wants answers. So we, we do not see both, both the sides try, uh, giving, uh, I mean trying, trying their best to, uh, give justice to a particular topic, right?
Johannes Castner:So what would you like your, uh, the, um, audience to take away from, from this discussion? And also, um, how would they keep in touch with you and follow your research?
Srijit Panja:Yeah, so I mean, you can, and I'm very much active on LinkedIn and that is the media I like. So, um, you can all reach out to me on LinkedIn, very much available. Uh, not an issue, uh, follow my research. Uh, Published my articles on, uh, I mean, whatever research I do that is on research gate. Um, so that is available. And from message perspective, uh, I, I, what, what I like to say is to, to remain hopeful. To, to remain hopeful always, and, uh, to, to, at least I like to see things not on a very surface level, but, um, to, on a, on a very deep, deep scientific level. So to try to analyze everything possible, uh, on, on a scientific, from a scientific perspective.
Johannes Castner:This show is published every Wednesday at 10:00 AM in the
United Kingdom, 5:00 AM on the East Coast, 2:00 AM on the West coast. Please leave us comments and tell us what you like the direction of the show. If you like the direction, if you don't like the direction, if you want to see something in particular on the show, please tell us. Subscribe if you haven't already, so that you can keep current with the show and give us as many comments as possible. And also give us a thumbs up on things that you like.